下载中心
优秀审稿专家
优秀论文
相关链接
首页 > , Vol. , Issue () : -
摘要
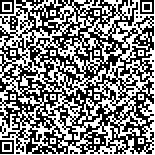
遥感影像由于成像特点和空间分辨率的制约,导致小目标特征提取难度增加,因此遥感影像小目标检测具有较大挑战。现有深度学习目标检测网络架构多基于自然图像而构建,在顾及遥感影像小目标特殊性方面的研究和探索存在不足。为此,本文提出了联合双重注意力机制和双向特征金字塔的遥感影像小目标检测算法。主要创新工作体现在:1)针对遥感影像中待检测物体所占比例较小导致其特征信息不易提取以及网络参数量大等问题,基于YOLOv3网络模型,引入LKGNet主干网络和GIoU损失函数,提出LKGNet-YOLO网络;2)针对网络特征提取过程中容易受噪声信息干扰以及网络多层信息融合能力不足的问题,基于YOLOv3网络模型,引入DA-LKGNet主干网络和加权双向特征金字塔结构,提出DA-LKGNet-YOLO网络。采用2014年中国科学院大学发布的遥感影像数据集UCAS-AOD v1.0(UA)和2021年武汉大学发布的AI-TOD数据集进行实验,结果表明:本文方法明显优于YOLOv3、RFBNet、SSD、FSSD、RetinaNet、RefineDet, mAP精度分别高出约3.45%-7.52%、1.36%-4.84%。同时,在小尺度目标上的检测水平优于Faster-RCNN和YOLOv7算法。与原始YOLOv3网络相比,本方法在浮点运算次数上降低了48%,参数量减少了42%。
Due to the imaging characteristics and the limitations of spatial resolution, feature extraction for small target is very hard, which increases the difficulty of small target detection. The existing deep learning target detection network architectures are mostly based on natural images, and it is insufficient in research and exploration of small target in remote sensing images. To overcome the above issues, this paper proposes a small target detection method based on improved YOLOv3. The innovative contributions are: 1) Aiming to sovle the problem of small target occupytion in the remote sensing image and the usually huge parameter size, we introduce LKG bottleneck and GIoU loss function into YOLOv3, and propose LKGNet-YOLO network. 2) Aiming to sovle the disturbance of noise and the drawback of feature fusion, we introduce DA-LKGNet bottleneck and BiFPN into LKGNet-YOLO, and propose DA-LKGNet-YOLO network. In order to verify the effectiveness of the proposed method, the remote sensing image dataset (UCAS-AOD) released by the University of Chinese Academy of Sciences in 2014 and the AI-TOD dataset released by Wuhan University in 2021 is used for experiments. The experimental results show that, compared to YOLOv3, RFBNet, SSD, FSSD, RetinaNet and RefineDet, the mAP accuracy of the proposed method is 3.45%-7.52% and 1.36%-4.84% higher, achieving significant performance improvement. Meanwhile, the detection level on small-scale targets is better than Faster-RCNN and YOLOv7 algorithms. Compared with the original YOLOv3, this method reduces the number of floating-point operations per second by 48%, and the number of parameters is reduced by 42%. The above experiments verify the effectiveness of our method.