下载中心
优秀审稿专家
优秀论文
相关链接
首页 > , Vol. , Issue () : -
摘要
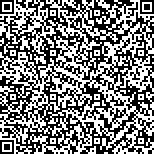
点云简化是海量机载LiDAR地面点云高效传输和多尺度应用的前提。针对目前地面点云简化方法存在复杂环境适用性差、地形细节特征丢失等问题,本文提出了一种顾及地形特征的机载LiDAR点云聚类简化算法。首先利用K-means算法将点云分割为初始点云簇,然后依据各簇的地形复杂度再次对其细分,接着借助点云法向量信息以及邻接簇间边缘点的高程差识别地形特征点,最后通过保留各簇的边缘特征点防止原始点云边界收缩。选取6组高密度机载LiDAR点云为数据源,将本文方法与七种经典点云简化方法(包括随机方法、体素格网方法、基于曲率的方法、最大Z容差方法、基于图的方法、基于聚类和基于迭代的简化方法)比较分析。实验结果表明:相同点云简化比例下,本文方法生成的数字高程模型(DEM)精度及其派生品(包括平均坡度和地形粗糙度)精度均明显优于传统方法,而且较好的保留了地形特征信息。
Point cloud simplification is a prerequisite for efficient transmission and multi-scale application of massive ground-based LiDAR ground point clouds. This paper proposes a LiDAR point cloud clustering and simplification algorithm that takes into account terrain features. The point cloud is first partitioned into initial clusters using the K-means algorithm, then subdivided into clusters based on the terrain complexity information of each cluster, followed by the identification of terrain features with the help of point cloud normal vector information and the elevation difference of edge points between neighbouring clusters, and finally the original point cloud boundary is prevented from shrinking by retaining the edge features of each cluster. Six sets of high-density example airborne LiDAR ground point clouds are selected for the study, and the new method is compared with the random method, the voxel grid method, the maximum Z-tolerance method, Curvature-based method, graph-based methods, clustering-based and iteration-based. The results show that the accuracy of the digital elevation model (DEM) generated by the new method and its derivatives (including mean slope and terrain roughness) are significantly better than those of the traditional method at the same point cloud simplification scale, and the terrain feature information is better retained.