下载中心
优秀审稿专家
优秀论文
相关链接
首页 > , Vol. , Issue () : -
摘要
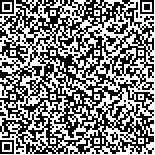
建筑物是城市中最为普遍的基础设施,获取遥感影像中的建筑区域对于城市规划、人口估计和灾情分析等具有重要的意义。本文基于Transformer结构,设计了一种端到端的遥感影像建筑区域提取方法。首先,针对多尺度影像特征存在的信息冗余和信息差异问题,本文提出了一种多次特征金字塔结构Tri-FPN,实现跨越近邻尺度的全局多尺度信息融合,提高多尺度特征的类别表征一致性并减少信息的冗余。其次,针对多尺度提取结果融合时仅考虑尺度因素的问题,本文设计了一种顾及“尺度-类别-空间”的注意力模块CSA-Module,有效融合了不同尺度下的建筑提取结果。最后,在Transformer结构上加入Tri-FPN与CSA-Module进行模型训练,获得最佳的建筑提取效果。实验对比分析表明,本文的方法有效提高了建筑区域的检出率,并提供出更为准确的建筑轮廓,提升了遥感影像中建筑的提取精度,在WHU Building数据集和INRIA数据集上分别取得了91.14%和81.6%的IOU分数。
(1)Objective:As the develop of deep learning, researchers are paying more attention to its application in remote sensing building extraction. In order to obtain better details and overall effects, many experiments about multi-scale feature fusion, which boosts the performance during the feature inference stage , and multi-scale outputs fusion are conducted to achieve a trade-off between accuracy and efficiency. However, current multi-scale feature fusion methods only consider the nearest feature, which is not sufficient for cross-scale feature fusion. The functions of multi-scale outputs fusion are also limited in an unary correlation, which only takes the scale element into account. To address these problems, we propose a feature fusion method and a results fusion module to improve the accuracy of building extraction from remote sensing images. (2)Method:This paper has proposed Tri-FPN(Triple-Feature Pyramid Network) and CSA-Module (Class-Scale Attention Module) based on Segformer to extract building in remote sensing images. The whole network structure is divided into three components: feature extraction, feature fusion and classification head. In the feature extraction component, this paper adopts the Segformer structure to extract multi-scale feature. The Segformer utilizes the self-attention function to extract feature maps of different scales. To adaptively enlarge the receptive fields, Segformer uses strided convolution kernel to shrink the key and value vector in self-attention computation process. The calculation cost decreases significantly. In the feature fusion component, the goal is to fuse the multi-scale feature from different part of the feature extraction network. Tri-FPN consists of 3 feature pyramid networks. The fusion follows a sequence of “top-down”, “bottom-up” and “top-down”, which enlarges the scale-receptive field. The basic fusion block are 3×3 convolution with feature element-wise addition and 1×1 convolution with channel concatenation. This design helps maintain the spatial diversity and the inner-class feature consistency. In the classification head component, each pixel is assigned a predicted label. First, the feature map goes through a 1×1 convolution to get a coarse result. Second the feature map shrinks in the channel dimension by 1×1 convolution. Third, the shrunk feature map is concatenated with the coarse result and 2× up-sampled. Fourth, the mixed feature is segmented by 5×5 convolution. A Height×Width×classes attention map, which takes class information, scale diversity and spatial details into account, is calculated by a 3×3 convolution block on the mixed feature at the same time. Last, the coarse result and the mixed-feature result is fused under attention map. (3)results:A series of experiments were carried out on the WHU Building and INRIA datasets.For the WHU Building dataset, the precision reaches 95.42%, the recall 96.25% and IOU 91.53%. For the INRIA dataset, the precision, recall and IOU reach 89.33%, 91.10% and 81.7% respectively. Compared with the backbone, the increase in recall and IOU exceed over 1%. It is proved that the proposed method has strong feature fusion and segmentation ability. (4)Conclusion:The Tri-FPN effectively improves the building extraction accuracy and the overall efficiency, especially on the boundaries and the holes in building area, which verifies the validity of multi-scale feature fusion. By taking C(class), S(Scale) and spatial attention into account, the CSA-Module can greatly improve the accuracy with negligible parameters. By adopting both Tri-FPN and CSA-Module, the structure improve the ability of predicting small buildings and the details in remote sensing images.