下载中心
优秀审稿专家
优秀论文
相关链接
首页 > , Vol. , Issue () : -
摘要
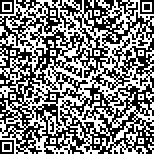
采空区地表裂缝识别对矿区的生产安全和生态环境治理具有重要意义。裂缝检测可以通过语义分割和边缘提取任务实现。已有的深度学习裂缝检测方法通常将其分开处理,无法为后续的几何参数计算和损害程度量化提供足够数据,并且没有考虑到两个任务之间的互补性。本文设计了一个双任务卷积神经网络Goaf-DTNet(Dual-Task Convolutional Neural Network for Goaf Crack Recognition)来同时获取裂缝面状分割和线性踪迹提取结果,通过建立两个任务之间的信息互补来提高模型的精度。在Goaf-DTNet的面状分割分支中,使用了多尺度特征融合提取鲁棒性特征。同时,在裂缝线性踪迹提取分支中,使用面状分割特征对线性踪迹特征提取过程进行引导,提高模型对裂缝的定位精度。为了验证方法的有效性,分别在自建的地表裂缝数据集和公共数据集上进行了实验,实验结果显示本文方法优于其它对比方法。消融实验结果也表明联合训练两个任务可以提升彼此的准确率。除此之外,在真实大场景图像上的实验结果表明本文方法在矿区地表裂缝监测中具有一定的实际应用潜力,可以为矿区监测提供有效数据。
Automatic detection of ground cracks in the goaf of coal mines plays an important role in production security and ecological environment management. Due to the complex background in the coal mine, the variable geometry and scale of cracks. Automatically detecting ground cracks in the goaf remains a challenging task. Cracks extraction could be treated as a segmentation task from a global view or a skeleton extraction task (boundary detection task) from a local view. There has been a lot of work view cracks extraction as a single task independently. However, those methods cannot produce enough data for subsequent measurement and quantitative evaluation about the extent of detriment of ground cracks. And they neglect the information interaction of different tasks which could potentially improve the accuracy and efficiency. In order to solve these problems, a dual-task convolutional neural network (CNN), named Goaf-DTNet (Dual-Task Convolutional Neural Network for Goaf Crack Recognition) is designed to automatically detect ground cracks using unmanned aerial vehicle (UAV) imagery with high spatial resolution. In Goaf-DTNet, an ASPP (atrous spatial pyramid pooling) module was introduced to extract multi-scale semantic information. Considering the characteristics of ground cracks in the goaf, MFFM (Multi-scale feature fusion module, MFFM) in the crack segmentation branch was designed to further integrate local and global contextual information. In order to improve the accuracy, in the crack skeleton extraction branch, SGFM (Segmentation-guided crack skeleton feature extraction module) was used to provide more spatial information through spatial attention mechanism. The proposed dual-task model can explicitly avoid calculating parameters in the shared layers, thus reduce the memory footprint and speed up each task. Meanwhile, through the communication between two tasks, the complementary information could improve the detection accuracy of each task. In the task of skeletion extraction, the F1-score and IoU (intersection over uinon) is 0.71 and 0.55 respectively, whiech is about 1% higer than the second best method, and the IoU is 16.35% higher than PSPNet. In the task of surface segmentaion, the proposed model perform best over all compared methods, the ODS(optimal dataset scale) and AP(averge precision) value is 0.56 and 0.54 respectively. In addition, the test results in a open-source dataset present that our method is better than the others, the F1, IoU value is 0.89 and 0.80 respectively. In terms of skeletion extraction, the OIS(optimal image scale), ODS, AP is 0.58, 0.58 and 0.55, respectively. In order to prove the effectiveness of the proposed MMFM and SGFM, ablation experiments were conducted. Results of ablation experiments show that, after adding MMFM in the crack segmentation branch, F1 and IoU increase 0.0827 and 0.0918 respectively, which reveals that it is helpful for crack detection through fusing the local and global information. In terms of crack skeleton extraction task, OIS, ODS and AP increased by 0.1246, 0.1630 and 0.2140 respectively after the SGFM was embedded into the branch. The experimental results show that the Goaf-DTNet is effective in ground cracks detection from the goaf of coal mines. MFFM proposed in this paper is conducive to the model to obtain more complete and continuous crack identification results by integrating multi-scale context semantic information. SGFM uses information from the surface segmetation branch to provide more spatial information for linear crack features, which effectively improve the detection accuracy. Furthermore, Taking advantage of the synergy between the two tasks , the accuracy of each task is imporved. Key words: Coal mines, goaf, unmanned aerial vehicle (UAV), crack detection, convolutional neural networks (CNNs), multi-task learning, deep learning