下载中心
优秀审稿专家
优秀论文
相关链接
首页 > , Vol. , Issue () : -
摘要
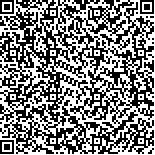
作者 | 单位 | 邮编 |
王濮 | 中国科学院空天信息创新研究院 | 100094 |
王成* | 中国科学院空天信息创新研究院 | 100094 |
习晓环 | 中国科学院空天信息创新研究院 | |
聂胜 | 中国科学院空天信息创新研究院 | |
杜蒙 | 中国科学院空天信息创新研究院 |
杆塔自动识别是机载LiDAR(Light Detection and Ranging)电力巡检应用的重要内容,特别是长距离、规模化应用时,高效高精度的杆塔点云提取尤为重要。针对复杂地形环境下输电通道杆塔点云快速精准识别难的问题,本文提出了一种基于多特征约束的杆塔自动识别方法。首先,基于输电通道地物空间分布特点,设计了离地高度、垂直最大间隙等特征;其次,对输电通道机载LiDAR点云进行去噪、滤波等一系列预处理;然后,对非地面点云进行网格化,基于离地高差、线性度等多特征约束快速定位杆塔区域,并利用分层密度法和杆塔塔体结构对称性提取杆塔中心坐标;最后,对杆塔区域点云垂直分层切片,逐层剔除非杆塔点云。采用三种不同场景的机载点云数据进行算法验证,结果表明本文所提方法可从原始点云中快速自动识别杆塔,其中查准率、召回率、F1值可达0.916、0.960、0.935,杆塔定位精度保持在分米级甚至厘米级。
Automatic identification of pylons is essential to airborne light detection and ranging (LiDAR) power inspection. The efficient and high-precision extraction of pylon point clouds is crucial, especially in long-distance and large-scale applications. This paper presents an automatic pylon point cloud identification method based on multi-feature constraints for the rapid and accurate identification of transmission corridor pylon point clouds under a complex terrain environment. First, the spatial distribution characteristics of the transmission corridor objects are analyzed, and the characteristics, such as the height above the ground and the maximum vertical gap, are designed. Second, some preprocessing operations, such as denoising and filtering, are performed on the airborne LiDAR point cloud of the transmission corridor. Third, the non-ground point cloud is gridded, and the pylon region is quickly located based on multi feature constraints such as ground elevation difference and linearity. The layered density method and pylon body structural symmetry are employed to extract pylon center coordinates. Finally, the non-pylon point clouds are eliminated layer by layer by vertical slicing. Airborne LiDAR data in three different scenarios are utilized for verification. Results indicate that the proposed method can quickly and automatically identify the pylon from the original point cloud. The precision, recall and F1 values can reach 0.916, 0.960 and 0.935 respectively. The pylon positioning accuracy is maintained at the decimeter or even centimeter level.