下载中心
优秀审稿专家
优秀论文
相关链接
首页 > , Vol. , Issue () : -
摘要
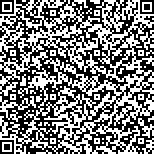
高成本与有限范围的实地监测已经无法满足植被物候研究的要求,而遥感物候监测方式又经常受到卫星传感器的时空分辨率等限制,这些局限使得图像融合成为高精度植被物候反演的关键。本研究基于Google Earth Engine(GEE)平台,以四个PhenoCam物候相机所观测的水稻、落叶林、玉米和灌木为研究对象,利用ESTARFM(Enhanced Spatial and Temporal Adaptive Reflectance Fusion Model)算法融合Landsat8影像与MODIS产品,生成了2018年1d、3d、5d、7d、9d、11d的30m遥感EVI时间序列,并采用Savitzky-Golay滤波和Maximum Separation方法提取生长季开始期(Start of Season,SOS)、结束期(End of Season,EOS)、生长季长度(Length of Season,LOS)等物候信息。我们发现:(1)与实测物候对比,整体上呈现时间分辨率越高,物候误差越低的趋势,且当时间分辨率小于7d时,融合物候的误差基本处在同一水平;(2)融合影像与Landsat8影像的空间特征基本一致,空间效率(Spatial Efficiency,SPAEF)指标为0.14-0.74,其中水稻、灌木与实际的空间一致性偏低;(3)融合结果与实地观测到的时间变化趋势吻合(RMSE: 0.01-0.02,r: 0.73-0.95),可以反演出较为准确的物候参数,SOS、EOS、LOS的平均误差为4.25d、4.75d、7.5d;(4)与MODIS物候反演结果相比,非农用地(落叶林和灌木)的物候参数误差缩小较为明显,而农业用地(水稻和玉米)的提升效果相对较小。本研究从空间和时间维度验证了ESTARFM算法生成的高时间分辨率EVI序列的可靠性,评估了其在物候监测能力上相比MODIS数据的提升效果,并探讨了影响融合效果的因素,可为精细化的植被动态监测和生态系统研究提供理论支撑和数据参考。
Vegetation phenology is referred to be the most direct and sensitive indicator for assessing the environmental changes. Currently, costly and limited in-situ observations at field scales are impractical for phenology monitoring. Alternatively, various aspects of phenology have been successfully characterized by remote sensing images. Due to the trade-off between spatial and temporal resolutions of satellite sensors, image fusion becomes the breakthrough for deriving fine-resolution phenological metrics. Most existing studies have retrieved phenology with sparse time series (with intervals > 8 days), consequently failing to capture the small intra-annual variations in phenology. Additionally, few scholars used relatively dense time series, but obtained less robust results. Thus, it is necessary to extract phenological metrics from fused results at finer temporal resolutions, and comprehensively validate them to expand our knowledge on the fusion method. In this study, we implemented a spatiotemporal fusion called ESTARFM (Enhanced Spatial and Temporal Adaptive Reflectance Fusion Model) on the Google Earth Engine (GEE) platform. By leveraging it, we generated 30m time series of EVI with different resolutions (1d, 3d, 5d, 7d, 9d, 11d) by fusing Landsat8 and MODIS images in 2018. We selected four PhenoCam sites in north America, of which the predominant vegetation types are paddy rice, deciduous forests, corn and shrubs (hereinafter referred to Cam1-Cam4, respectively). And we used Savitzky-Golay filtering and Maximum Separation method to estimate phenological metrics such as Start of Season (SOS), End of Season (EOS), Length of Season (LOS). We evaluated the effect of temporal resolutions on the phenometrics derived from the fusion results with field data (from PhenoCam project). Then we used the daily fused time series for further illustration. The fused images were validated by true Landsat8 observations with sufficient unmasked pixels. Finally, we used MODIS data to assess the improvement of accuracy of derived phenometrics fusion results. We generated 133, 273, 126, 288 images by ESTARFM at daily resolution at Cam1-Cam4, respectively. These fused images obtain more spatial phenological details than lower-resolution images (e.g. MODIS), and the errors of phenometrics derived from fusion results generally increase along with temporal resolutions. And the errors become rather more obvious when the time resolution reaches 7d. We conduct research and discussions under daily resolution: the correlational results prove that fused images can accurately capture reliable spatial patterns with SPAEF (Spatial Efficiency) ranging from 0.14 to 0.74, and are temporally consistent with field observations (RMSE: 0.01-0.02, r: 0.73-0.95). The derived phenological metrics are relatively accurate, with mean errors of 4.25d, 4.75d and 7.5d for SOS, EOS, LOS, respectively. Comparing with MODIS data, ESTARFM reduces the errors of fusion-derived phenometrics for deciduous forests and shrubs evidently. This research deployed a new framework on the GEE platform to estimate phenological metrics derived from satellite images fused by ESTARFM and assessed the accuracy with field data in North America. Our findings revealed the reliability of the EVI time series with fine resolutions generated by ESTARFM. This framework helps us to obtain more authentic details of spatiotemporal phenological distributions. We are sure our study will provide important supportive theories and data basis for the issues concerning vegetation dynamics and ecosystem.