下载中心
优秀审稿专家
优秀论文
相关链接
首页 > , Vol. , Issue () : -
摘要
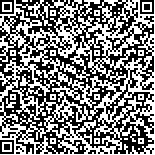
目的 遥感影像由于雾霾的原因导致清晰度下降,增添了遥感影像目标检测和地物分割任务的困难。方法 提出一种基于自适应SLIC的遥感影像去雾算法,主要包括:首先,针对有雾遥感影像出现局部区域高亮问题,使用改进的Retinex算法,对输入遥感影像进行对比度增强,保留图像细节,以减少伪影现象,扩展图像的对比度动态范围,准确估计遥感影像大气强度值;其次,提出了一种自适应SLIC算法,解决超像素数目参数设定的困难,对输入遥感影像进行超像素分割,避免局部对比度强烈区域对固定窗口的影响,从而获得更加精确的透射率估计;最后,基于暗通道先验原理和大气散射模型恢复出无雾遥感影像。结果 使用所提算法和He等人、Zhu等人、Han等人和Nie等人的四种算法进行对比,分别在公开数据集Inria Aerial Image Dataset和RICE Image Dataset进行去雾效果比较。主观上,所提算法处理后的遥感影像颜色更真实、去雾更彻底、地物更清晰,能更好的保留影像细节信息;客观上,所提算法处理后的图像信息熵平均值为7.56,峰值信噪比平均值为22.05,结构相似性平均值为0.87,均高于其他四种算法。结论 所提出的去雾算法模型,综合了图像增强与恢复的优点,使得去雾后的遥感影像更加自然真实,更好的恢复出遥感影像细节信息。
Objective Remote sensing images are degraded in clarity due to haze, which adds difficulties to remote sensing image target detection, feature segmentation tasks and remote sensing image information interpretation. The remote sensing image defogging method based on deep learning is time-consuming due to the large number of model parameters and the dependence on the amount of remote sensing image data. The remote sensing image dehazing method based on image enhancement does not fully consider the degradation mechanism of remote sensing images in hazy, which makes it difficult to adapt to remote sensing im-ages in different scenes and easily loses image information leading to image distortion. The remote sensing image dehazing method based on physical model requires manual setting of parameters when refining the transmittance, and at the same time, as the contrast of remote sensing images is not completely enhanced, the overall colour of the dehazed images is dark and the fog remains in local areas. Method To solve the above problems and improve the quality of remote sensing image dehazing, a re-mote sensing image dehazing method based on image enhancement and physical modeling is proposed. An adaptive SLIC-based remote sensing image dehazing algorithm is proposed, which mainly includes: Firstly, for the problem of local area highlighting in hazy remote sensing images, and and the problem of atmospheric intensity value calculation bias problem, an improved Retinex algorithm is used to contrast-enhance the input remote sensing images to preserve image details in order to reduce artifacts, extend the dynamic range of image contrast, and accurately estimate the atmospheric intensity value of remote sensing images. Secondly, an adaptive SLIC algorithm is proposed to solve the difficulty of setting the parameter of the number of superpixels and perform superpixel segmentation on the input remote sensing image to avoid the influence of the local con-trast intensity region on the fixed window, to obtain a more accurate transmittance estimation. Finally, a haze-free remote sens-ing image is recovered based on the dark channel a priori principle and atmospheric scattering model. The proposed method can achieve adaptive dehazing of remote sensing images without manual parameter setting. Results The proposed algorithm is compared with the four algorithms of He et al., Zhu et al., Han et al. and Nie et al., and the dehazing effects are compared in the publicly available datasets Inria Aerial Image Dataset and RICE Image Dataset, respectively. Subjectively, the remote sensing images processed by the proposed algorithm have more realistic color, more complete dehazing, clearer features, and can better retain image detail information. Objectively, the mean value of image information entropy, peak signal-to-noise ratio and struc-tural similarity of the proposed algorithm is 7.56, 22.05, and 0.87, which are higher than the other four algorithms. Conclusion The proposed dehazing algorithm model integrates the advantages of image enhancement and recovery, which makes the dehazed remote sensing images more natural and realistic and better recovers the remote sensing image detail information.