下载中心
优秀审稿专家
优秀论文
相关链接
摘要
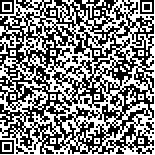
水利设施对水资源与水量调度、自然湿地生态保护与修复、资源和生态功能的利用及经济效益发展有重要作用。传统方法统计水利设施位置、数量等依赖于汇编资料,存在耗时长、资料更新不够及时以及具体地理位置不详等缺点,遥感为大规模监测水利设施提供了新的可能。本文以YOLO v3网络为基础,结合水利设施的特点,提出了一种基于大面幅影像快速检测水利设施的算法,主要分为两个方面:(1)改进的YOLO算法(E-YOLO)。E-YOLO提出PPA特征融合方法和等比预测框与四特征图交叉预测方法,对小样本等问题进行优化;改进损失函数,突出置信度损失;同时使用迁移学习的方法,读取特征提取部分的预训练模型参数。(2)基于E-YOLO算法和水体指数约束的大面幅水利设施检测算法。通过水体指数约束滑动步幅来解决影像面幅大、目标尺度小的问题,同时降低漏检率和误检率,再结合轮廓合并方法,优化检测结果。本研究中采用高分二号影像数据实现大面幅影像水利设施检测,实验结果表明:E-YOLO算法可以明显提高水利设施检测效果,相比平均F2精度相比YOLO v3提高了1.25%。且有更好的稳定性;水体指数约束的大面幅检测方法可以在保证效率的情况下提高检测精度,其F2精度相比大步幅和小步幅方法分别提高了3.72%和2.70%,为遥感水利设施检测提供了良好方案。
Water facilities play an important role in water scheduling, ecological protection and restoration of natural wetlands, utilization of resources and functions, and development. The traditional methods of statistics on the location and count of water conservancy facilities rely on compiled data, which has disadvantages such as time-consuming, untimely data update, and unknown specific geographic locations. Remote sensing provides new possibilities for large-scale detection of water conservancy facilities. Aiming at the problem of detection of water conservancy facilities with remote sensing images, this study proposes a large-scale image detection algorithm. Based on the YOLO v3 network and the characteristics of water conservancy facilities, the study was divided into two main aspects: (1) We improved the YOLO algorithm and obtained the E-YOLO algorithm. We proposed a PPA feature fusion method and a four-feature map cross prediction method with proportional prediction box to optimize the problems of small samples. Besides, we improved the loss function by highlighting the loss of confidence. In addition, we used the transfer learning method to read part of the feature extraction parameters of the pre-trained model. (2) With the improved E-YOLO algorithm as the core, a large-area water conservancy facility detection algorithm combined with the water body index constraint was obtained. Aiming at the problem of large image size with a small target scale, we used the water body index to constrain the sliding step to reduce the missed detection rate and false detection rate at the same time. Then we combined the network output with the contour merging method to optimize the detection results. We used the GF-2 data for this study. The experimental results show that: the E-YOLO algorithm can significantly improve the detection effect of water conservancy facilities. Compared with YOLO v3, the average F2 score of E-YOLO is increased by 1.25% and the E-YOLO algorithm has a better stability. The large-area detection method constrained by the water index can improve the detection accuracy while ensuring efficiency. Compared with the large-step and small-step methods, its F2 accuracy is increased by 3.72% and 2.70%, respectively. Our method provides a good solution for the detection of water conservancy facilities.