下载中心
优秀审稿专家
优秀论文
相关链接
摘要
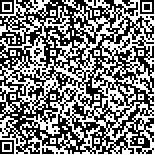
太赫兹频段在冰云探测上具有独特优势,但是目前的太赫兹冰云反演算法将不同种类冰相粒子(主要是冰和霰)视为冰粒子统一计算。本文根据冰云太赫兹辐射特性实现了一种预分类的神经网络算法,能够从太赫兹亮温中分别反演得到冰、霰两种粒子的统计参数和廓线分布。首先,基于WRF数值模式和ATMS载荷真实观测的冰云霰数据构建了包含冰、霰粒子密度廓线的混合冰云数据库,然后,使用DOTLRT辐射传输模式模拟183 GHz、243 GHz、325 GHz、448 GHz、664 GHz和874 GHz这6个频段的星载太赫兹冰云探测亮温,最后,开展冰云参数探测仿真试验,验证反演算法性能。仿真试验中反演得到的冰和霰的路径总量均方根误差分别为8.97 g/m2和10.90 g/m2,等效粒径均方根误差分别为7.54 μm和25.38 μm,反演的冰、霰密度廓线也具有较高的精度。研究结果表明本文算法能够以较好的精度从多频太赫兹冰云探测亮温数据中分别反演得到冰、霰两种粒子的路径总量、等效粒径、等效云高和密度廓线,突破现有研究仅仅计算单一冰粒子的局限,更加符合冰云真实情况。
Terahertz band has a number of potential advantages that complement existing visible and infrared techniques in ice cloud sounding application, but treating various phase ice particles (mainly ice and graupel) as single ice particles is a major limitation of current terahertz ice cloud retrieval algorithms. In this paper, a pre-classified neural network algorithm based on the terahertz radiation characteristics of ice cloud is proposed, which is able to retrieve the physical parameters of ice and graupel particles, respectively. The algorithm first uses a pre-classified neural network to retrieve the density profiles of graupel particles separately from the 183 GHz band brightness temperature data. The retrieved graupel profiles are then used as a priori constraint to calculate the higher frequency band brightness temperature difference due to ice particles only. Finally, another pre-classified neural network is used to retrieve the density profiles of ice particles separately from the preceding terahertz brightness temperature difference data. The proposed algorithm are evaluated through the end to end simulation experiments. Firstly, a hybrid ice cloud dataset including ice and graupel particle parameters is built based on the numerical weather prediction (NWP) model and the actual observation data. Then the synthetic ice cloud brightness temperature data from 183—874 GHz (i.e. 183 GHz, 243 GHz, 325 GHz, 448 GHz, 664 GHz and 874 GHz) are generated through Discrete-Ordinate Tangent Linear Radiative Transfer (DOTLRT) radiative transfer model with the hybrid ice cloud dataset. Finally, the parameters of ice and graupel are retrieved by the proposed algorithm from the simulated brightness temperature data, and compared with the input parameters to assess the retrieval accuracy. The simulation experiments show that the average Root Mean Square Errors (RMSE) of the retrieved IWP and GWP are 8.97 g/m2 and 10.90 g/m2 respectively, and the average RMSE of the retrieved I_Dme and G_Dme are 7.54 μm and 25.38 μm respectively, and the average RMSE of the retrieved I_Zme and G_Zme are 309.21 m and 513.62 m respectively, and the retrieved density profiles of ice and graupel particles also have high accuracy. The results indicate that the proposed algorithm can retrieve the total path amount, equivalent ice particle size, and equivalent ice cloud height and density profile of ice and graupel particles respectively with high accuracy, which is more consist with the real condition of ice cloud than the current retrieval algorithm.