下载中心
优秀审稿专家
优秀论文
相关链接
摘要
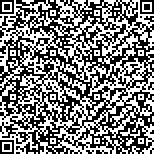
小样本学习旨在利用非常少的监督信息识别出新的类别,由于忽视了样本之间的关联信息,现有的小样本分类方法用于遥感图像小样本分类时往往不能获得令人满意的精度。为此,本文利用图来建模图像在特征空间的相似关系,使用图卷积运算平滑同类别图像的特征,增强不同类别图像特征的区分度,提升分类精度。所提方法在现有图卷积运算的基础上,使用多阶次的邻接矩阵线性加权的方法代替传统的一阶邻接矩阵,通过图谱分析得出这种改进方法能够让不同阶次邻接矩阵的频率响应函数在高频部分正负相抵,有效抑制图信号的高频分量,更显著的提升同类别节点特征的聚集程度;同时,在训练过程引入了微调的方法,使用新类别中的标记数据对最后一层图卷积网络进行少量次数的训练,能够进一步提高精度,增强模型的迁移能力。实验使用AID、OPTIMAL31以及RSI-CB256这3个常用的遥感数据集对方法的有效性进行了测试,结果表明提出的方法在同数据集小样本分类任务和跨数据集小样本分类任务中,在分类精度方面均优于原型网络等比较方法。
Few shot classification is a hot topic in the field of deep learning, which aims at identifying novel concepts with little supervisory information. In the remote sensing (RS) scene few shot classification, existing methods are often unable to achieve satisfactory accuracy because the relationships among samples are ignored. To improve the RS few shot classification accuracy, the multi-graph convolutional network (Multi-GCN) is proposed in this paper. In the proposed method, a graph convolutional network is introduced into the metric network to smooth the samples’ features, which can model relationships among samples, make images from the same class get more similar feature representation and improve the classification accuracy. The proposed Multi-GCN is mainly composed of three parts: (1) Feature extraction network, which is composed of 4-layer convolutional neural networks and is used to extract images’ features; (2) Graph convolutional network, which is used to model the relationships among samples in the feature space, and update the node features by multi-graph convolution; (3) Metric prediction part, which is used to calculate the prototype of each class, and predict labels of the unlabeled samples according to the distance between samples and prototypes. Based on spectral domain analysis, the proposed multi-graph convolution can effectively suppress the high-frequency components of the graph signals and significantly enhance the clustering coefficients of features from the same class. In addition, the fine-tuning method is introduced in the training process, and labeled samples in the new class are used to train the last layer of the graph convolutional network for a small number of steps, which can further improve the classification accuracy and enhance the transfer ability of the model. To validate the effectiveness of the proposed method, in the experiments, Multi-GCN is compared with ProtoNet, GNN-FSL, and two single graph based methods ProtoGCN and ProtoIGCN on two different tasks, i.e. the same dataset few shot classification task and cross datasets few shot classification task. From the experimental results, we can obtain that in the same dataset few shot classification task, the proposed method is significantly superior to ProtoNet. When the number of labeled samples of each class is 1, the accuracy of the proposed method is higher than ProtoNet by more than 5%; Compared with GNN-FSL, the accuracy of the proposed method is about 10% higher on average. In the cross datasets few shot classification task, the classification accuracy of the proposed method is 10%—15% higher than that of GNN-FSL; And compared with ProtoNet, it is about 10% higher in the case of 1-shot and about 2%—5% higher in the case of 5-shot. In most cases, Multi-GCN is also better than single graph methods, such as ProtoGCN and ProtoIGCN. The classification accuracy can be further improved by using fine-tuning training methods. From the above experimental results, we can conclude that the proposed method can achieve a higher classification accuracy compared with ProtoNet, GNN-FSL, and the methods based on a single graph convolutional network. The conclusions of multi-graph convolutional operation and spectrum analysis in this work can also be extended to other graph-based semi-supervised learning.