下载中心
优秀审稿专家
优秀论文
相关链接
摘要
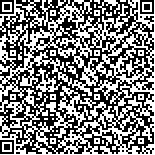
确定森林火烧迹地的准确时间点以及空间范围对于森林的受损评价、管理、碳核算以及森林恢复的管理有重要意义。由于森林火烧迹地在空间分布上具有一定的连续性,现有的森林火烧迹地提取方法大都采用先分类再后处理的两步处理策略来抑制虚警像素的影响。本文提出将时空检测方法Stacked ConvLSTM用于时间序列森林火烧迹地的检测,在保持结果具有较好空间连续性的基础上避免了具有主观性的后处理操作,实现端到端提取森林火烧迹地信息,提升了森林火烧迹地的提取精度。采用MODIS时间序列数据,基于2001年—2008年以及2001年—2016年的黑龙江沾河林业局伊南河林场和内蒙古自治区毕拉河林业局北大河林场两个区域的历史时间序列,分别对这两个区域2009年以及2017年发生的特大火灾区域进行火烧迹地检测,利用Stacked ConvLSTM、Stacked LSTM以及bfast算法在两个区域的MODIS时间序列中提取森林火烧迹地,并将火烧迹地检测结果与ESA发布的Fire_CCI 5.1火烧迹地产品进行对比分析。结果表明:首先,从目视效果来看,在研究区域Ⅰ,Stacked ConvLSTM检测的结果比Stacked LSTM和bfast算法错误检测点少,并且在空间分布也保持较高连续性;在研究区域Ⅱ,Stacked ConvLSTM检测到了较完整的火烧迹地区域。其次,在定量的精度评价指标上,在研究区域Ⅰ,Stacked ConvLSTM的精确度比Stacked LSTM和bfast算法分别高出0.120和0.405,并且召回率、准确度和F1-score也更高,Fire_CCI 5.1召回率虽更高,由于错检区域较大,其他精度指标远低于Stacked ConvLSTM;在研究区域Ⅱ,Stacked ConvLSTM精确度达0.924,召回率、准确度和F1-score相比Stacked LSTM和bfast算法以及Fire_CCI 5.1更高。
关键词:
Stacked ConvLSTM 时间序列 时空预测 火烧迹地As the largest land cover, forests play an important role in human living environment, biological habitat, and global carbon cycle. Forest health is directly related to global ecological security and sustainable development of human society. In recent years, urban construction, disasters, forest management and deforestation, and other factors have caused different degrees of disturbance to forests. It is important to determine the exact time point and spatial range of forest burned area for forest damage assessment, management, carbon accounting, and forest restoration management. Owing to the continuity of spatial distribution of forest burned areas, most of the existing methods of forest burned area extraction use the two-step treatment strategy of first classification and then post-processing to suppress the effect of false alarm pixels. In this paper, a spatiotemporal detection method, Stacked ConvLSTM, is proposed for the detection of forest fire tracks in time series. This method avoids subjective post-processing operations on the basis of maintaining better spatial continuity of the results, and achieves end-to-end extraction of forest burned area information, which improves the extraction accuracy of forest fire-burning land. This paper proposes to use Stacked ConvLSTM to detect forest disturbance in time and space. Combined with the characteristics of ConvLSTM in extracting temporal and spatial characteristics from long-term historical series, it can predict the change trend of vegetation in a period of time in the future, and accurately determine the time point and spatial range of forest disturbance. ConvLSTM is an LSTM variant proposed on the basis of LSTM. The full connection state from input layer to hidden layer and from hidden layer to hidden layer of LSTM is replaced by convolution connection, which can make full use of spatial information. Compared with single-pixel-based methods, ConvLSTM can extract the spatiotemporal structure information of time series images at the same time, which is better for spatiotemporal analysis. In this paper, Stacked ConvLSTM is used to detect the temporal and spatial distribution of forest burned areas, predict the change trend of vegetation in a period of time in the future, and determine the presence of forest burned areas by comparing with the newest time-series images. With MODIS long time series data, based on the historical time series of Yinanhe Forest Farm of Zhanhe Forestry Bureau in Heilongjiang Province and Beidahe Forest Farm of Bilahe Forestry Bureau in Inner Mongolia from 2001—2008 and 2001—2016, the extraction results of burned areas were compared with Stacked LSTM and bfast algorithm. The Stacked ConvLSTM, Stacked LSTM, and bfast algorithms were used to extract forest burned areas from MODIS time series in both regions, and to compare the detection results with the Fire_CCI 5.1 burned areas products released by ESA. Results show that, firstly, from the visual effect, in study area Ⅰ, the error detection of Stacked ConvLSTM is fewer than that of Stacked LSTM and bfast algorithm and maintains high continuity in spatial distribution. In study Area Ⅱ, Stacked ConvLSTM detected a more complete area of fire. Secondly, in study area Ⅰ , Stacked ConvLSTM was 0.120 and 0.405 more accurate than Stacked LSTM and bfast algorithms, respectively. Moreover, the recall rate, accuracy, and Fire_CCI 5.1 F1-score were higher. In study area Ⅰ , the accuracy of Stacked ConvLSTM is 0.924 had a higher recall rate, accuracy, and F1-score than Stacked LSTM, bfast algorithms, and Fire_CCI 5.1. The detection accuracy of ConvLSTM model in space is higher than that of the other two methods, and its continuity of detection results in space is better. The detection effect of ConvLSTM model is equivalent to that of Stacked LSTM in time, but both of them are closer to the real fire time point than bfast algorithm. Results show that Stacked ConvLSTM has advantages in obtaining the change trend of forest long-term historical series for spatiotemporal prediction, and improves the detection accuracy of forest fire to a certain extent.