下载中心
优秀审稿专家
优秀论文
相关链接
摘要
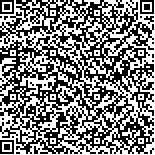
城市建成环境是人类赖以生存的人造环境,城市建成环境存量是指城市中建筑物和基础设施的材料质量。反演城市建成环境存量的空间分布,是数字城市建设的新方向,它能够帮助我们了解城市发展模式,更加有效管理城市资源和废弃物等,对发展城市循环经济、实现城市的可持续发展有着十分重要的意义。本文详细介绍了城市建成环境存量空间计算的3种方法(自上而下、自下而上和遥感计算方法)的理论基础和发展现状,总结了目前的几中方法都存在着过度依赖统计数据、无法兼顾研究区域大尺度和高空间分辨率等问题。在地理大数据时代,更多的数据源为存量的计算带来了新的研究方向。本文总结了新数据源的优势,并展望了结合地理大数据和机器学习方法的存量计算方法,为城市建成环境存量的空间计算提供了一种新的思路。
Urban built environment is the manufactured environment where human beings live. The stocks of the urban built environment refer to the quality of materials (e.g., concrete, steel, copper, etc.) that accumulated in buildings and infrastructure. Revealing the spatial distribution of urban built environment stocks arises as a new direction for digital city construction, which helps to understand the urban development patterns and urban resource and waste management. Developing an urban circular economy and realizing sustainable urban development is essential. Therefore, it is necessary to summarize and sort out the current spatial calculation method of built environment stocks.This study introduces a detailed theoretical basis and development status of three methods for spatial calculation of urban built environment stock: that are the top-down method, the bottom-up method, and the remote sensing calculation method. The advantages and limitations of these models are elaborated with application and data availability. The top-down approach has a complete set of theoretical foundations and algorithm models, which can perform large-scale material flow analysis well. Due to its inability to obtain a high spatial resolution, this method is not suitable for analyzing urban development within cities. Contrastingly, the bottom-up method permits fine-grained stock estimation by gathering cadastral-level physical measurements of buildings and infrastructure and associated material composition indicators. However, it is labour-intensive and the scope of the bottom-up method is often restricted to city-level or lower geographical regions. As for remote sensing calculation, previous studies established a linear regression relationship between the nighttime light radiation intensity and the built environment stocks in the study areas. However, the night light remote sensing data will degrade the reliability of quantitative analysis due to background noise and radiation saturation effect. Thus, stock data with the high spatial resolution are impossible to acquire. These three traditional methods are often difficult to strike a balance between large scale and high spatial resolution. However, in the era of big geographic data, more data sources have brought new research directions for stock calculation.Geo Big Data and Earth Observation data are essential in developing earth science, environmental science, remote sensing science, and geographic information science. Combining these wide-coverage, high-precision, and fast-update data and machine learning methods have been widely used in poverty surveys and energy consumption. This paper proposes a framework that combines big geographic data and machine learning for stock calculation based on the above background. We expect an end-to-end method to estimate grid stocks directly from publicly available information that minimizes manual involvement. However, the heterogeneity of geospatial and the black-box nature of deep learning may have an impact on the migration effects of the model. Despite its drawbacks, this migration model has the potential for large-scale, high-resolution stock calculation in future works.