下载中心
优秀审稿专家
优秀论文
相关链接
首页 > , Vol. , Issue () : -
摘要
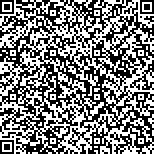
叶片叶绿素含量(Leaf chlorophyll content,ChlLeaf)是反映植被光合能力强弱的重要指标,对全球碳循环研究和农业监测有重要意义。高分六号(GF-6)搭载的宽幅相机具有两个对ChlLeaf敏感的红边波段,具备开展ChlLeaf定量反演的潜力。本文提出基于叶绿素含量敏感指数CSI(Chlorophyll sensitive index)的GF-6宽幅相机WFV(Wide Field of View)数据ChlLeaf反演方法,通过PROSAIL和PROSPECT+4-Scale模型模拟构建了ChlLeaf与CSI指数的经验回归模型,并与MTCI、CIre和TCARI/OSAVI等红边指数的经验回归模型的反演精度进行对比。基于农作物、阔叶林和针叶林三种植被类型实测数据的验证结果表明,CSI指数反演精度最优(R2 = 0.62, RMSE = 10.31 μg cm-2),高于CIre指数(R2 = 0.34, RMSE = 14.83 μg cm-2)、MTCI指数(R2 = 0.25, RMSE = 15.3 μg cm-2)和TCARI/OSAVI指数(R2 = 0.01, RMSE = 21.34 μg cm-2)。利用GF-6 WFV数据生成2019年北京森林站ChlLeaf时间序列与现有MODIS ChlLeaf产品的对比结果表明,GF-6能提供更高分辨率的ChlLeaf数据,能够更准确地刻画ChlLeaf的空间和时间序列变化特征,可以为GF-6在农业和植被生态监测的研究和应用提供数据支撑和科学依据。
Chlorophylls are the dominant pigments in plant photosynthesis. The leaf chlorophyll content (ChlLeaf) is directly related to the photosynthetic capacity and plays an important role in global carbon cycle modeling and agricultural monitoring. Gaofen-6 (GF-6) satellite is China’s first high-spatial-resolution satellite for precision agriculture. The GF-6 wide field of view camera (WFV) with a 4-day revisit cycle and 16-meter spatial resolution has two red-edge bands that are sensitive to variations in ChlLeaf and shows great potential for ChlLeaf monitoring at fine temporal-spatial resolution. However, few studies focusing on vegetation parameters quantitative inversion from GF-6 WFV data and the applicability of GF-6 WFV for ChlLeaf retrieval is still yet to be validated. In this study, we proposed a ChlLeaf retrieval algorithm for GF-6 WFV based on chlorophyll sensitive index (CSI) and constructed a CSI-based empirical regression model by the relationship between ChlLeaf and CSI using PROSAIL and PROSPECT+4-Scale model simulations. The inversion accuracy of CSI-based empirical regression model was then compared with other vegetation indices-based empirical regression models such as MTCI, CIre, TCARI/OSAVI. First, the PROSAIL model and PROSPECT+4-Scale model were used to generate simulated canopy reflectance of croplands, broadleaf forests and needleleaf forests, respectively, and the canopy reflectance simulations were resampled to GF-6 WFV multispectral reflectance using the spectral response function of GF-6 WFV. Then, CSI derived from simulated GF-6 WFV reflectance was used to construct the CSI-based empirical model for ChlLeaf retrieval by regression analysis. Finally, the accuracy of CSI-based retrieval model was evaluated using ground-measured ChlLeaf data and the existing MODIS ChlLeaf product. The results showed that CSI was more linearly related to ChlLeaf and less sensitive to LAI variations than MTCI, CIre and TCARI/OSAVI. CSI achieved better ChlLeaf retrieval accuracy with R2 = 0.62 and RMSE = 10.31 μg cm-2, higher than CIre (R2 = 0.34, RMSE = 14.83 μg cm-2), MTCI (R2 = 0.25, RMSE = 15.3 μg cm-2), TCARI/OSAVI (R2 = 0.01 and RMSE = 21.34 μg cm-2). Under different LAI and ChlLeaf conditions, the variations of CSI-based model in RMSE were the lowest, which suggested that CSI offered a more stable approach to retrieval ChlLeaf than the other three vegetation indices. The comparison of the GF-6 WFV ChlLeaf time-series and the MODIS ChlLeaf product at the Beijing forest site indicated that GF-6 WFV could provide high spatial resolution ChlLeaf dataset, which was capable of deriving information on ChlLeaf variations at finer temporal-spatial resolution. In conclusion, the GF-6 WFV data have good potential for accurate retrieval of ChlLeaf at regional scales. The CSI-based GF-6 ChlLeaf can achieve high retrieval accuracy and portray the spatial and time series variation characteristics of ChlLeaf, which provides the data support and scientific basis for the further research and application of GF-6 WFV in agriculture and vegetation ecological monitoring.