下载中心
优秀审稿专家
优秀论文
相关链接
摘要
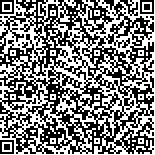
对被飓风破坏的森林进行变化监测与灾害评估是遥感技术的一个重要应用,遥感影像的特征信息提取对森林遥感监测的效果至关重要。多样性特征结合可以有效提高对森林变化的监测精度。然而,当前的空间信息如纹理特征的获取算法依旧保留着传统的固定式计算模式,一直面临着特征数量和邻域参考范围之间难以均衡的问题。为了解决以上问题,本文提出了基于多样性特征协同技术的飓风前后森林破坏遥感监测方法,首先计算出森林遥感影像变化前后的归一化植被指数差值和增强植被指数差值,并提出了基于复合窗口技术的来提取纹理特征,然后建立了多样性特性结合模型;其次提出了一种基于特征分离的旋转森林改进算法,最终,实现了内泽尔森林在暴风前后的高精度变化监测;另外,还测试了新模型在不同训练样本数量下的分类性能。实验结果表明,相对传统的基于光谱特征和单纯的纹理特征的变化监测方法,本文所提出的方法的整体精度、对变化区域和未变化区域的检测精度至多分别提高了3.68%、6.53%和3.46%。本文的研究方法可以有效提高森林变化监测的性能,为森林灾害评估与森林资源保护提供参考依据。
Change monitoring and disaster assessment of hurricane-damaged forests are important applications of remote sensing technology. The extraction of feature information from remote sensing images is very important to forest remote sensing monitoring. The combination of diversity features can effectively improve the accuracy of forest change monitoring. However, the current spatial information acquisition algorithms, such as texture features, still retain the traditional fixed computing model and do not fully consider the diversity of the spatial distribution of ground objects. When extracting texture features, the accuracy of texture features is affected when the sliding window is extremely large or small. Therefore, the calculation method of this kind of texture feature is always faced with the problem of hard balance between the number of features and the neighborhood reference range. Therefore, this paper focuses on forest change monitoring technology. To solve the above problems, a remote sensing monitoring method of forest destruction before and after hurricanes are proposed based on diversity features collaborative technology. First, the difference between the normalized vegetation index (NDVI) and the Enhanced Vegetation Index (EVI) before and after forest remote sensing image change is calculated. Second, the compound window technology is proposed to extract the texture features. Then, the texture features extracted from the remote sensing image and the spectrum of the remote sensing image are used to build a diverse characteristic combination model. This model can enhance the diversity of features. Finally, an improved rotating forest algorithm based on feature separation is proposed to reduce the direct feature correlation and improve the accuracy of the classifier. The study area is the remote sensing images of the Nezer forest in France before and after the hurricane, which were obtained from the Formosat-2 satellite. In the experimental part, the classification performance of the proposed algorithm was compared with those of six other methods. Experimental results show that compared with the traditional change detection methods based on spectral and texture features, the overall accuracy of the proposed method, the detection accuracy of the changed area, and the unchanged area improved by 3.68%, 6.53% and 3.46%, respectively. In addition, the sensitivity of the features extracted by different methods to the number of training samples was tested. The results show that the proposed method maintains high classification accuracy on different training sample numbers, and its overall accuracy and Kappa coefficient are better than those of the comparison methods. After the number of training samples reaches 50, the accuracy of the proposed method tends to flatten. The proposed method can effectively improve the performance of forest change monitoring. This method can be used to monitor the change of and damage to forests in real time and efficiently obtain information on forest disaster areas, providing important reference data for the emergency decision-making of forest resource management departments. Therefore, this method has a high practical value.