下载中心
优秀审稿专家
优秀论文
相关链接
摘要
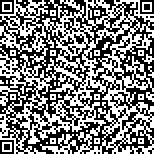
遥感图像目标检测是遥感图像处理中的一个基本问题,尤其是伴随着深度学习以及遥感影像获取等技术的发展与突破,基于深度学习的遥感图像目标检测得到了广泛的关注。区别于自然图像,遥感图像中的物体目标具有方向任意的特点,众多国内外学者提出一系列基于倾斜边界框的遥感图像目标检测算法,推动了遥感图像目标检测的提升。为使得相关领域研究者对基于深度学习的遥感图像倾斜框目标检测的理论、流程及其现存问题有一个比较全面的认知,本文将对其进行系统的整理和归纳。
Object detection is a fundamental aspect of remote sensing image processing. With the development of remote sensing image acquisition and the breakthrough in deep learning, object detection in aerial imagery based on deep learning has attracted considerable interest. Although significant progress has been made, there are still numerous obstacles due to the large-scale and highly complex backgrounds of optical remote sensing images. In addition, approaches based on horizontal proposals for common object detection frequently suffer from the mismatch issue when detecting densely arranged and arbitrarily oriented objects in aerial imagery. Therefore, numerous domestic and international researchers have proposed tilting box object detection algorithms based on deep learning that enhances the object detection effect of remote sensing images. This paper systematically organizes and summarizes them for researchers in related fields to comprehensively understand the theory, process, and existing problems of deep learning-based remote sensing image tilting box object detection.In this paper, we first analyze the limitations of Horizontal Bounding Box (HBB) object detection algorithms applied to remote sensing images, namely, the introduction of background noise, inappropriate post-processing operation, Non-Maximum Suppression (NMS), and the inability to accurately determine the orientation of objects, which can be remedied by the tilting bounding box object detection method.Following this, we list the classical HBB object detection algorithms based on deep learning and briefly describe their underlying principles. Then, the development of the tilting bounding box object detection algorithm and the process of improving the two-stage tilting bounding box object detection algorithm is described from three perspectives: the feature extraction network, anchor boxes, and the proposed region design. Finally, the one-stage detection algorithm’s loss function has been studied infrequently, so the two algorithms are merely introduced.In the fourth section, the detection performance of existing tilt box object detection algorithms is demonstrated on two publicly available and challenging aerial datasets (i.?e., DOTA and HRSC2016). The comparison results of the three tables indicate that a particular object feature enhancement module must be designed to account for the uniqueness of the objects in remote sensing images and that the RSE problem in the algorithm for detecting tilting bounding boxes requires additional consideration. Although the one-stage detection algorithm is marginally less accurate than the two-stage algorithm, it has clear advantages in terms of efficiency and therefore has some research value.The paper concludes with a six-point summary of the tilt box target detection algorithm’s existing problems and an outlook on its future development trend.