下载中心
优秀审稿专家
优秀论文
相关链接
摘要
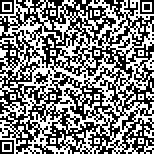
基于深度神经网络模型的遥感影像地物检测取得了巨大成功,很大程度上得益于大规模数据集的支撑。但是,从现有遥感影像数据集本身来看,不同类别地物的数量分布不一致,同类地物对象以不同尺寸大小呈现,是导致地物样本的尺度不均衡问题的直接因素。对此,本文采用数据集内影像加权融合与地物多尺度特征选择的策略来缓解该问题。首先,将数据集内两张影像的像素值进行加权并得到融合后的影像,从而使不同类别地物样本更加均衡且具有较高的背景多样性;其次,通过选择合适尺度的特征图预测相应尺度的目标类别,且允许同一尺度目标在相邻特征图上进行预测,这样使模型能根据目标尺度进行训练;最后,基于目标中心区域的特征图预测目标边界框,预测的边界框更符合目标本身的尺度。通过在两个遥感数据集上分别进行实验,表明训练的模型在对复杂背景下的类别不均衡目标的识别更加准确,能够适应遥感影像下不同尺度目标的识别。
Remote sensing image object detection based on depth neural network model has achieved great success largely due to the support of large-scale data sets. However, from the perspective of the existing remote sensing image datasets, the number distribution of different types of ground objects is inconsistent, and the same type of ground objects is presented in different sizes. This factor leads to the scale imbalance of ground object samples.To alleviate this problem, the strategy of weighted image fusion and multiscale feature selection is adopted. First, the pixel values of the two images in the data set are weighed to obtain the fused image. Therefore, the different types of feature samples are more balanced and have higher background diversity. Second, the target category of the corresponding scale is predicted by selecting the appropriate scale feature map, and the same scale target can be predicted on the adjacent feature map. Thus, the model can be trained according to the target scale. Finally, the bounding box of the target is predicted based on the feature map of the target center area, and the result is more consistent with the scale of the target itself.The image fusion and multiscale feature selection network is experimentally compared in the paper on two datasets, RSOD and NWPUVHR10. The results show that the trained model is more accurate in the recognition of imbalanced objects in complex background and can adapt to the recognition of different scale objects in remote sensing images. In addition, the proposed method enhances the diversity of sample scenes and can be adapted to different scales of targets. Qualitative analysis shows that the proposed method has better robustness and performance advantages when applied to remote sensing images.The proposed method can cope with remote sensing images with complex backgrounds, mitigate the effects of category imbalances and better fit the scenarios in which remote sensing images are used. Mitigation of category imbalances and Scale Selection enhance the performance of remote sensing image object detection.