下载中心
优秀审稿专家
优秀论文
相关链接
摘要
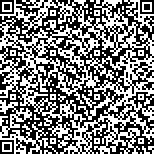
高分辨率遥感影像建筑物自动提取在防灾减灾、灾害估损、城市规划和地形图制作等方面具有重要意义。但是,目前常用的传统卷积神经网络模型存在异变性强而同变性弱缺陷。针对该问题,本文提出一种基于通道和空间双注意力胶囊编码—解码网络DA-CapsNet(dual-attention capsule encoder-decoder network)的建筑物提取通用模型。该模型通过胶囊卷积和空间—通道双注意力模块增强高分辨率遥感影像中建筑物高阶特征表达能力,实现建筑物遮挡部分以及对非建筑不透水层的准确提取与区分。模型首先利用胶囊编码—解码结构提取并融合多尺度建筑物胶囊特征,获得高质量建筑物特征表达。之后,设计通道和空间注意力特征模块进一步增强建筑物上下文语义信息,提高模型性能。本文选取3种高分辨率建筑物数据集进行试验,最终的平均精度、召回率和F1-score分别为92.15%、92.07%和92.18%。结果表明,本文提出的DA-CapsNet能有效克服高分辨率遥感影像中的空间异质性、同物异谱、异物同谱以及阴影遮挡等影响,实现复杂环境下的高精度建筑物自动提取。
Automatic extraction of buildings from high-resolution remote sensing images is greatly important in disaster prevention and mitigation, disaster loss estimation, urban planning, and topographic map making. With the advancement of optical remote sensing techniques in image resolutions and qualities, remote sensing images have provided an important data source for assisting the rapid updating of building footprint database. Despite the large number of algorithms proposed with enhanced performance, fulfilling highly accurate and fully automated extraction of buildings from remote sensing images is still difficult due to the considerable challenging scenarios of buildings, such as color diversities, topology variations, occlusions, and shadow covers. Thus, exploiting advanced and high-performance techniques to further improve the accuracy and automation level of building extraction is greatly meaningful and urgently required by a large variety of applications.To overcome the issues of strong variability and weak homogeneity of traditional convolutional neural networks, we propose a novel dual-attention capsule encoder–decoder network DA-CapsNet for extracting buildings. In this network, a deep capsule encoder–decoder network, along with the channel-spatial attention blocks, is developed to enhance the capability of extracting high-level feature information from very high resolution remote sensed images. Thus, this model has the ability to extract buildings covered by shadows and discriminate buildings from non-building impervious surfaces. Specifically, we initially employ a deep capsule encoder–decoder network to extract and fuse multiscale building capsule features, resulting in a high-quality building feature representation. Moreover, spatial attention and channel attention modules are designed to further rectify and enhance the captured contextual information to obtain a competitive performance in processing buildings in the diverse challenging scenarios. The contributions include the following: (1) the deep capsule encoder–decoder network is designed to generate a high-quality feature representation; (2) the channel and spatial feature attention modules are designed to highlight channel-wise salient features and focus on class-specific spatial features.The proposed DA-CapsNet was evaluated on three datasets: one Google Building Dataset and two publicly-available datasets (Wuhan and Massachusetts). The experimental results achieved a competitive performance with an average precision, recall, and F1-score of 92.15%, 92.07%, and 92.18%, respectively, in handling buildings of varying challenging scenarios. Considering the overall accuracy of F1-score, the DA-CapsNet achieved the values of 92.70%, 94.01%, and 89.84% for Google, WUH, and MA datasets, respectively. Comparative studies also confirmed the robust applicability and superior performance of the DA-CapsNet in building extraction tasks.