下载中心
优秀审稿专家
优秀论文
相关链接
摘要
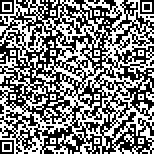
为了提高遥感图像中多尺度飞机目标的检测精度,本文提出一种基于改进Faster R-CNN的遥感图像飞机目标检测方法。该方法借助多层级融合结构,将深层次的语义特征与浅层次的细节特征相结合,生成多种尺度的既具有精确的位置信息又具有深层次的语义特征的特征图;再借助Faster R-CNN的多尺度RPN(Region Proposal Network)机制,通过对RPN中候选区域尺度的修正,从而提高遥感图像中多尺度飞机目标的定位精度;最后利用Faster R-CNN的分类回归网络,得到飞机目标检测结果。在高分辨率遥感图像中进行了实验,对3种特征提取网络ZF、VGG-16以及ResNet-50进行改进,改进后的精度分别提高了11.34%、9.87%以及1.66%,并且生成的检测框更加贴合飞机目标。实验结果表明,本文方法适用于遥感图像多尺度飞机目标检测,在提高目标定位精度的同时降低了目标漏检现象。
关键词:
遥感图像 目标检测 Faster R-CNN 多层次融合结构 多尺度Aircraft detection from optical imagery is a significant application in remote sensing. Traditional methods based on corner points or shape of the aircraft can only generate shallow features with limited representative ability. These methods are insufficient for detecting aircraft in remote sensing imagery under complex and diverse circumstances. Current methods based on CNNs, especially Faster R-CNN, have improved the detection performance greatly with its magnificent feature extraction ability. However, detecting aircraft on a single-scale feature map is unsuitable for multiscale aircraft in remote sensing imagery. After several pooling operations on a single-scale feature map, the feature map loses its precise details and small target that corresponds to a smaller area in the feature map. Thus, aircraft detection may result in low target positioning accuracy and target missing.An advanced Faster R-CNN is presented by constructing a multiscale feature extraction network using multistage fusion structure to detect aircraft with multiple scales. The promoted network produces features of higher resolution by upsampling deep feature maps. These features are then enhanced with shallow features at the same scale. After this modification, we end up with four feature maps F2, F3, F4, and F5, which have different scales. The structure combines the high-level semantic information with the low-level detailed information. Thus, the generated multiscale feature maps have high positioning accuracy and good distinguishability. In addition, because the original RPN anchors are extremely large to cover the range of aircraft sizes in remote sensing imagery, we select suitable RPN anchor parameters for aircraft detection, i.e., anchor size of 322 for the larger-scale feature map F2, 642 for the large-scale F3, 1282 is set for the F4, and 2562 for the small-scale F5. With these settings, the RPN can generate proposals, which can cover the aircraft of multiple scales. Finally, these proposals are assigned to their corresponding feature map, and we use the classification and regression network to obtain our final detection results.The experiment was carried out on RSOD dataset, in which only the aircraft dataset was used for training, validation, and testing. Comparison of detection performance with different anchor scales showed that anchor scales greatly affect detection accuracy, and our selection of anchor scales is suitable for the dataset. Three feature extraction networks (ZF, VGG-16, and ResNet-50) were modified based on Faster R-CNN using multistage fusion structure. The experiment showed that the modification can effectively improve the model’s ability of detecting multiscale aircraft. Compared with models without the modification, AP increased by 11.34%, 9.87%, and 1.66% for the three networks. The qualitative and quantitative results also showed that this modification can generate adaptive detection box. The experiment results on Beijing Capital International Airport GF-2 imagery showed that this method performs well in different remote sensing imagery, in which most airplanes in the airport were detected successfully.We can draw the following conclusions: (1) the proposed method is suitable for multiscale aircraft detection, and it can generate detection box consistent with the scale of multiscale aircraft targets while reducing missing targets; (2) correction of the RPN candidate region scale improves the accuracy of aircraft detection in remote sensing imagery; (3) the method has good generalization ability.