下载中心
优秀审稿专家
优秀论文
相关链接
摘要
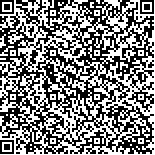
针对森林火灾模型模拟、预测火灾蔓延结果精度随时间增加而降低的问题,提出了使用卫星遥感火监测数据重初始化火灾模型降低模拟结果误差的方法。使用FARSITE(Fire Area Simulator)火灾模拟系统模拟内蒙古自治区一场森林火灾,并使用375 m的VIIRS(Visible infrared Imaging Radiometer Suite)火监测数据对FARSITE重初始化,探究该方法在中国林火蔓延模拟中的应用。使用SC(S?rensen’s Coefficient)值,辅以多源卫星遥感数据,评估该方法模拟结果精度。结果显示,各模拟过程中,模拟结果精度均随模拟时间的增加而降低,重初始化的模拟结果与实际过火区一致性更高,VIIRS重初始化模拟结果SC值最高提高56.89%,最终SC值提高了45.45%;最终模拟结果的SC值从最初的54.14%经过VIIRS火点修正后提高到78.76%;模拟过程中的VIIRS火点重初始化的模拟结果SC值最高为87.8%。使用VIIRS火监测数据重初始化FARSITE火灾模拟系统,将火灾分为多次进行模拟,缩短模拟时间,有效控制模拟误差的传递,提高了森林火灾蔓延模拟的精度,这种方法提高了火灾模型在大规模、长时间森林火灾应用中的可靠性。
Forest fires seriously affect the environment and social economy, e.g., damaging infrastructure, causing economic losses, and endangering human health. Effective simulation and prediction of forest fire growth are greatly important. Fire behavior models can provide analytical schemes for characterizing and predicting the speeds and directions of fire spread. However, fire spread models are subject to assumptions and limitations that inherently produce compounding errors during simulations. Satellite remote sensing monitoring of forest fire can be used to analyze the spatial dynamic change process of large-scale fires. It is an economical and effective technology for obtaining fire information in a large range and a short period. It can also provide fire location information for fire spread models.This study proposes a new approach of fire spread simulations based on the assessment of simulated fire growth discrepancies by using satellite active fire data. The FARSITE fire spread simulator was used to simulate the spread of forest fires that occurred on May 17, 2017 in Chenbaerhuqi, Inner Mongolia autonomous region, China, and the S-NPP\VIIRS forest active fire data were applied into the FARSITE simulator for calibration and re-initialization. The Landsat-8 and GF-1 data were used to generate the data required by the FARSITE. The fire field for different time periods was monitored by the multisource satellite data Sentinel-2A, GF-1 and GF-4 data. 375 m VIIRS active fire monitoring data were employed for re-initializing FARSITE fire simulation. We combined the satellite fire data and fire spread model for reducing errors of simulation results caused by the condition limitation of fire model, and the S?rensen’s coefficient (SC) was employed to evaluate the accuracy of fire spread simulation results at FARSITE before and after reinitializing the simulator for VIIRS active fire data.The re-initialization results of the FARISTE simulator by VIIRS active fire data showed that the simulation accuracy in each simulation process gradually decreased along with time. The distribution of simulation results indicated that the simulation findings after re-initialization were consistent with the actual fire perimeter monitored by high or moderate resolution remote sensing data. The highest precision in the process using active fire data increased by 56.89%, and the final accuracy increased by 45.45%. The final SC value increased from 54.14% to 78.76% when the satellite data were used to re-initialize the FARSITE fire simulation system, with increment of 42.76%. The maximum SC value was 87.8% for VIIRS active fire data re-initialization during simulation. The re-initialization approach meaningfully improved the accuracy of fire simulation.The use of satellite remote sensing active fire data and the re-initialization of FARSITE limited the further expansion of the error of fire spread model and improved the reliability and accuracy of forest fire simulation. This innovative approach represents a potential scheme for reducing the error of large-scale fire simulation results that can improve the reliability of fire spread model. This method provides an effective data assimilation method for fire prediction. It also provides a basis for fire management departments to manage forests and develop fire suppressing plans. In this study, the actual ground and air fire-fighting forces change the results of fire spread. They are an important factor for the deviation between the simulation results and the actual results.