下载中心
优秀审稿专家
优秀论文
相关链接
摘要
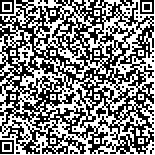
由于显著的经济效益和生态效益,近年来稻虾共作模式分布面积迅猛扩张。准确获取稻虾田空间分布信息,对于水稻种植结构调整、产量估算和水资源管理具有重要意义。本文以“小龙虾之乡”——湖北省潜江市为研究区域,基于Google Earth Engine平台协同Landsat 7/8和Sentinel-2卫星数据,通过分析稻虾田的农业耕作管理和季相节律特征,提取了稻虾田区别其他农作物的关键“水淹”信号和“植被”信号。基于实地稻虾田样本统计分析关键特征的阈值,构建稻虾田规则集识别模型,提取了湖北省潜江市2019年稻虾田空间分布。最后,基于实地样本验证该物候窗口特征方法的精度,并评估和比较了该方法与随机森林和基于水体季相差异方法的表现。结果表明:物候窗口1月1日—4月30日内的水淹信号(LSWI>NDVI或EVI)、物候窗口7月15日—9月30日内的植被信号(NDVI或EVI>LSWI)和物候窗口11月10日—12月31日内的水淹信号是稻虾田遥感识别的典型特征。基于该方法提取的2019年潜江市稻虾田制图精度和用户精度分别为90.74%、94.69%,显著高于水体季相差异方法和随机森林方法的精度。基于关键物候窗口的稻虾田提取方法具有较高的泛化能力,能以较少的实地样本进行时空尺度的延展,从而为大尺度长时序稻虾田遥感制图提供重要的方法支撑。
Rice-crayfish co-culture is a kind of comprehensive ecological agriculture pattern. Rice-crayfish co-culture has expanded rapidly in China in the past decade due to its outstanding ecological and economic benefits. The accurate spatial distribution information of this newly emerging agricultural pattern is crucial for growth monitoring, yield estimation, and water resource management. However, most studies have focused on field-level research on the farmland ecosystem, and rice-crayfish mapping at regional or larger scales has received less attention.In this study, Qianjiang City in Hubei Province, known as the “hometown of crayfish” was selected as the test area. The cloud computing approach was used for all available Landsat 7/8 and Sentinel-2 imagery in 2019 with the Google Earth Engine (GEE) platform. By analyzing farming characteristics and the spectral curves of rice-crayfish fields, we identified the crucial phenology windows and classification features (i.e., flooding and vegetation signals) for rice-crayfish mapping. On the basis of the key phenological characteristics and associated frequency thresholds derived from field samples, we developed a rule-based algorithm for rice-crayfish mapping and generated the rice-crayfish map of Qianjiang City in 2019. To further evaluate the potential of our proposed method, we compared it with the random forest method and a method based on seasonal differences of water bodies.The spectral analysis of time series images showed that the unique phenological characteristics of rice-crayfish co-culture were flooding signal (LSWI > NDVI or LSWI > EVI) in phenology window 1 (from January 1 to April 30), vegetation signal (NDVI > LSWI or EVI > LSWI) in phenology window 2 (from July 15 to September 30), and flooding signal in phenology window 3 (from November 10 to December 31). With the mapping results, the total area for rice-crayfish planting in Qianjiang City in 2019 was estimated to 575.58 km2, and rice-crayfish plots were mainly distributed in the southwest. The producer’s accuracy of the classification result was 90.74%, the user’s accuracy was 94.69%, and the overall accuracy was 95.23%. The method based on phenology window features had fewer commission errors compared with the random forest method and fewer omission errors compared with the method based on water body seasonal differences. Among the three methods, the proposed method presented the highest overall classification accuracy.The rice-crayfish mapping method based on phenology windows, flooding signal, and vegetation signal showed high separability. The method based on phenology windows can be easily generalized to other regions and other images because of its strong physical interpretation for rice-crayfish. On the one hand, this method has relatively low dependence on training samples. On the other hand, as long as the key phenology window can be obtained, other medium-high resolution images, such as GF-1 and GF-6, can achieve high-accuracy mapping results for rice-crayfish. Therefore, the method based on phenology windows can be effectively extended to large areas and long time series. It can provide essential information for rice production management and decision-making in the crayfish industry.