下载中心
优秀审稿专家
优秀论文
相关链接
摘要
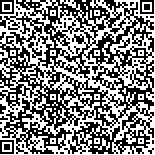
玉米和大豆是两种主要的粮食作物,及时准确地监测两者的种植面积对于产量预测和市场价格的制定具有重要的意义。利用遥感技术探究在生长季中后期能有效区分玉米和大豆的指示性特征集,为在不同实验区进行推广应用和提前玉米和大豆种植面积信息发布的时间提供技术支撑。文章以玉米和大豆为研究对象,以黑龙江和安徽省两个典型种植区为实验区,以高分一号影像为数据源,计算多种植被指数特征和两种纹理特征,同时利用特征优选方法评价特征间的相对重要性,并结合随机森林分类算法分析特征个数对精度的影响,得到不同试验区区分两者的最佳特征子集。随后根据不同实验区最佳特征子集的共同点和差异,遴选出对玉米和大豆中后期区分的遥感指示性识别特征集,并设计实验方案验证其有效性和稳定性。实验表明:在玉米和大豆生长中后期存在具有高效辨识两者的遥感特征集,能有效和稳定地增强两者的遥感识别能力;在不同实验区,基于特征优选方法可以选择出区分玉米和大豆的最佳分类特征子集,得到两者最优的识别效果,比仅仅使用原始波段特征的分类精度提升了近10个百分点,总体分类精度能够平均达到97%,Kappa系数0.96,玉米和大豆的单类分类精度平均超过95%;在不同的种植区,利用玉米和大豆的指示性特征集可以得到几乎与优选出的最佳特征子集同样的分类精度和制图效果,且具有稳定性和有效性,较最佳特征集更具推广使用意义。指示性特征集包含6种:植被指数中的比值植被指数(RVI),差值植被指数(DVI),转换型植被指数(TVI),改进型叶绿素吸收比率指数(MCARI)和灰度共生矩阵(GLCM)纹理特征中的二阶矩(the Second Moment)和熵(Entropy)。
Corn and soybean are two major crops maintaining food security, and thus the timely and accurate monitoring of their planting areas are of great importance to forecasting their production and market prices. The objectives of this study were to use a remote-sensing technology in exploring indicative features that can effectively identify corn and soybean in their middle and late growth seasons and provide technical support for the broad geographical application of corn and soybean mapping. This study can facilitate the early release of corn and soybean planting acreages for policy makers. In this study, two typical planting areas of corn and soybean in the provinces of Heilongjiang and Anhui were selected. GaoFen-1 satellite images with 30 m spatial resolution were acquired in the middle and latter growth stages and used as data sources for calculating various vegetation indices and textural features. Then, a feature optimization method was used in evaluating the relative importance scores of input features, and optimal feature combinations for identifying corn and soybean were determined. The random forest classification algorithm was used in analyzing the relationship between the number of input features and classification accuracy, and then the best feature groups in different experimental areas were identified. Finally, according to similarities and differences among the selected features in different regions, the indicative features for mapping corn and soybean in the middle and latter stages were established. The validity and stability were confirmed using our experimental designs. The following results were obtained: (1) indicative remote sensing features for efficiently identifying corn and soybean in their middle and late growing seasons were identified; (2) the classification performance of the indicative features of corn and soybean in both experimental areas was approximately 10% higher than that when original spectral band combinations were used. In different planting areas, high classification accuracy was obtained using the indicative features of corn and soybean as the optimal features selected in individual local area. Our selected indicative features for soybean and corn mapping were found stable, effective, and useful for large areas of implementation. These features included Ratio Vegetation Index (RVI), Difference Vegetation Index (DVI), Conversion Vegetation Index (TVI), improved chlorophyll absorption ratio index (MCARI), and the second moment and entropy in Gray Level Co-occurrence Matrix (GLCM).