下载中心
优秀审稿专家
优秀论文
相关链接
摘要
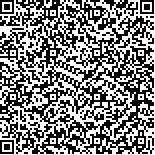
基于大数据驱动的深度学习挖掘图像数据的规律和层次已成为遥感影像解译的研究热点。海量标签样本是训练深度学习模型的前提条件,但成本昂贵的人工标记样本限制了深度学习技术在遥感领域的应用。本文提出了一种基于弱样本的深度学习模型农作物分类策略:以GF-1影像为数据源,将传统分类器SVM分类结果视为弱样本,训练深度卷积网络模型DCNN(Deep Convolutional Neural Networks),获取辽宁省水稻和玉米的空间分布,分析弱样本的适用性。结果显示:测试集总体精度达到0.90,水稻和玉米F1分数分别为0.81和0.90;在不同地形地貌、复杂种植结构的农业景观下均表现出良好的分类效果;与SVM结果的空间一致性为0.90;当弱样本最大面积误差比例小于0.36时,弱样本仍适用于DCNN作物分类,结果的总体精度保持在0.86以上。综上,该策略一定程度上消除了深度学习模型对大量人工标记样本高度依赖的局限性,为实现大尺度农作物遥感分类提供了一种新途径。
Driven by big data, deep learning has been widely and successfully applied in many fields, such as computer vision and speech recognition. With the increase in network depth, deep learning models can determine the rules and layers of images and obtain high classification accuracy, so they have become a research hotspot in remote sensing image interpretation. As data-driven algorithms, deep learning models need a large number of labeled samples for training to ensure that the trained model can learn accurate and comprehensive sample features, and they exhibit good classification performance. However, although the development and maturity of remote sensing technology provide abundant remote sensing image sources for deep learning models, the application of deep learning technology in remote sensing is limited by expensive manually labeled samples,. In practical crop classification applications, the quantity and quality of existing ground truth samples are often insufficient to train a classification model with high performance. This study proposes a crop classification strategy for the deep learning model based on weak samples to verify the applicability of the deep learning model with weak samples. GF-1 was used as the data source, and the SVM classifier was used to classify three types of rice, corn, and other ground objects in Liaoning Province at the county level. The results were used as the training label samples of the deep learning model. This process included sub-county SVM classification, manual post-classification processing, cropland masking, and other operations. This human-computer interaction was chosen to ensure the accuracy of the results. In this study, samples with non-100% accuracy were labeled as weak samples. Then, a Deep Convolutional Neural Network (DCNN) model was used to train the weak samples and obtain the spatial distribution of rice and corn in Liaoning Province. Results showed that OA reached 0.90, and the F1 scores of rice and corn were 0.81 and 0.90, respectively. The spatial consistency with the SVM results was 0.90. The model showed good robustness under the different topography and landform types of the agricultural landscape with a median OA that was greater than 0.93. It overcame the influence of topography in the study area to a certain extent through subregion analysis. In the agricultural landscape with a complex planting structure, the proposed method still maintained a certain accuracy in crop classification. Subsequently, noise experiments were designed to analyze the influence of SVM label noise on model classification. The corn distribution in the original SVM training label was expanded from 1 to 40 times to obtain new labels, which were then used to train the DCNN model and predict the testing data. When the model was within five times the sample noise, that is, the sample maximum error area ratio was not more than 0.36, the model was robust to a certain extent, and the results could be maintained within a reliable accuracy range (OA remained to be greater than 0.86). In conclusion, this study verified that crop classification results obtained with the deep learning model whose training labels are based on traditional classification methods can achieve high recognition accuracy good robustness under different topography and landform types of agricultural landscapes and the feasibility of using traditional classification results as weak samples. The experiment on increasing noise in the weak samples showed that weak samples can be used to train DCNN as long as their identification accuracy is guaranteed, that is, the maximum error area ratio of samples is not more than 0.36. This approach further reduces the threshold of obtaining labeled samples via deep learning models. It makes up for the limitation of the deep learning model, which is highly dependent on a large number of manually labeled samples, and provides a new approach for large-area remote sensing crop classification.