下载中心
优秀审稿专家
优秀论文
相关链接
摘要
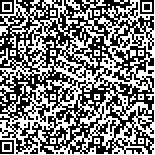
随着对地立体观测体系的建立,遥感大数据不断累积。传统基于文件、景/幅式的影像组织方式,时空基准不够统一,集中式存储不利于大规模并行分析。对地观测大数据分析仍缺乏一套统一的数据模型与基础设施理论。近年来,数据立方体的研究为对地观测领域大数据分析基础设施提供了前景。基于统一的分析就绪型多维数据模型和集成对地观测数据分析功能,可构建一个基于数据立方的对地观测大数据分析基础设施。因此,本文提出了一个面向大规模分析的多源对地观测时空立方体,相较于现有的数据立方体方法,强调多源数据的统一组织、基于云计算的立方体处理模式以及基于人工智能优化的立方体计算。研究有助于构建时空大数据分析的新框架,同时建立与商业智能领域的数据立方体关联,为时空大数据建立统一的时空组织模型,支持大范围、长时序的快速大规模对地观测数据分析。本文在性能上与开源数据立方做了对比,结果证明提出的多源对地观测时空立方体在处理性能上具有明显优势。
The volume of Earth Observation (EO) data has tremendously increased after the establishment of EO system. Managing such big EO data and turning them into valuable information is a major challenge in EO domain. This study proposes a multisource EO cube toward large-scale analysis.The infrastructure accommodates multisource geospatial data including raster and vector data. A cube model is designed, and four dimensions including product, space, time, and band dimension are formalized. Several cube explore examples are presented. The infrastructure enables large-scale analysis based on cloud computing technology, and a set of distributed cube objects extending Spark Resilient Distributed Dataset for cube tiles is designed. The distributed cube objects are compatible with multiple data source including raster and vector data. A multi-thread computing method is used together with cloud computing, which forms a hybrid parallelism, to further improve data access and processing efficiency. Batch computation is also used to address the issue that massive number of tiles cannot be loaded into memory at one time. Moreover, a machine learning-based approach is integrated into the cube to enhance parallel geoprocessing. The computational intensity of tiles can be predicted and saved in databases in advance, which eliminates the extra time cost of computational intensity prediction on the fly for those commonly used products. The design and implementation for the cube infrastructure, named GeoCube, is provided. It covers the ingestion and management of multisource geospatial data in the cube, the processing of geospatial/EO queries against different cube dimensions, and high-performance cube computing of large-scale geospatial datasets. The creation of such a geospatial data cube help advance the EO data cube approach while keeping connections to the data cube in the BI domain.The performance on data query and access, data processing, and load balance is presented. Results demonstrate the advantage of GeoCube infrastructure. Several applications are presented including cube OLAP operations, large-scale time-series analysis, and multisource data cube analysis.In conclusion, compared with existing cube approaches, the proposed infrastructure emphasizes the accommodation of multisource geospatial data including raster and vector data in the cube, cube tile processing with cloud computing, and artificial intelligence machine learning-enabled cube computation. Such a cube can inherit not only the large-scale processing capabilities of EO data cubes but also the data management capabilities of BI data cubes.