下载中心
优秀审稿专家
优秀论文
相关链接
首页 > , Vol. , Issue () : -
摘要
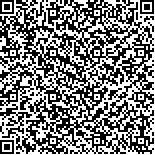
随着合成孔径雷达(SAR:Synthetic Aperture Radar)成像和深度学习技术的发展,利用深度学习算法对SAR图像进行地物分类得到了广泛关注及应用研究。本文基于对地观测航空遥感系统高分机载数据构建了高分辨率机载多维度SAR地物分类数据集(AIR-MDSAR-Map: Airborne Multi-Dimensional Synthetic Aperture Radar Mapping Dataset)。本数据集包含海南万宁和江苏射阳两个区域的P、L、S、C、Ka五个波段的多极化 SAR 图像和高分辨率光学图像,将地物划分为水域、裸地、道路、工业区、林草地、住宅区、种植地、养殖场和其他等9类,并提供精细化的像素级别标签。本文利用深度学习中较经典的语义分割方法对 AIR-MDSAR-Map 进行地物分类验证,同时也检验了不同波段SAR图像对各类地物的分类敏感性。最后,通过不同的融合策略对多维度SAR数据进行融合分类,最终融合方法在其中多类地物目标上的表现优于单波段,在综合指标FWIoU和PA上提升10~15%,FWIoU达69%,PA达81%。该数据集将发布于国家综合地球观测数据共享平台(http://www.chinageoss.cn),能满足不同行业用户和科研用户的需求,为多维度SAR数据的应用研究提供支撑。
With the development of Synthetic Aperture Radar (SAR) imaging and deep learning, the use of deep learning to classify land cover in SAR images has received extensive attention and applied research. In this paper, a high-resolution airborne multi-dimensional SAR land cover classification dataset is constructed based on the high-resolution airborne data of the Chinese Aeronautic Remote Sensing System (CARSS) for Earth observation, whose name is AIR-MDSAR-Map (Airborne Multi-Dimensional Synthetic Aperture Radar Mapping Dataset). The original data are obtained by CARSS, and the platform is a modified Xinzhou 60 remote sensing aircraft. SAR images and optical images are generated according to the standard data production process. After imaging processing, radiometric correction, polarization correction, and geometric correction, the original SAR data are preprocessed to form SLC data, and then geometric processing is used to generate SAR DOM products. After image enhancement, splicing, and rough correction, the raw optical data are preprocessed to generate DSM data, and then semi-automatic filtering is performed to produce DEM. Finally, AIR-MDSAR-Map contains polarization SAR images in bands of C, Ka, L, P, and S and high-resolution optical images in Wanning, Hainan, and Sheyang, Jiangsu, with the spatial resolution ranging from 0.2 m to 1 m depending on the band. AIR-MDSAR-Map divides the land cover into nine categories and generates fine pixel-level labels through a semi-automatic labeling algorithm. In this paper, the classical semantic segmentation methods in deep learning, such as UNet, SegNet, Deeplab, and HRNet, are used to verify the classification of AIR-MDSAR-Map. At the same time, we also test the classification sensitivity of different band images to all kinds of land cover objects. This dataset includes multi-dimensional SAR images of the same place and time, which can be used for fusion classification research. In this paper, multi-dimensional SAR data are fused and classified through different fusion strategies, model fusion classifies land cover by selectively fusing the models of each band, and the priori fusion uses the prior information of the classification results in each band to distinguish land cover on defining the priority of objects. These two fusion methods outperform the single-band in the performance of some types of land cover, and improve the FWIoU and PA by 10~15%, the FWIoU reaches 69%, and PA is 81%. AIR-MDSAR-Map can satisfy the research and application requirements of different users and can be used to study the characteristics of the same land cover object with different resolutions, bands, and polarizations. Moreover, it can provide a strong promotion for the development of multi-dimensional SAR applications. The AIR-MDSAR-Map will be available at the ChinaGEOSS Data Sharing Network (http://www.chinageoss.cn).