下载中心
优秀审稿专家
优秀论文
相关链接
首页 > , Vol. , Issue () : -
摘要
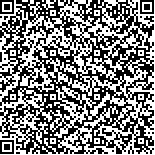
针对光学遥感图像中云的遮挡可能会降低甚至完全遮挡图像中的某些地面覆盖信息,限制对地观测、变化检测或土地覆盖分类等的问题,云去除是迫切需要解决的一项重要任务。为了恢复被云遮挡的地面区域,提出一种基于多时相遥感图像的两阶段云去除算法。第一阶段是云分割,即直接使用U-Net提取云并去除薄云。第二阶段是图像恢复,采用时空生成网络(STGAN)去除厚云,STGAN的生成模型采用改进的多输入的U-Net,通过一次从同一位置的7帧图像序列中提取关键特征恢复相应的不规则厚云覆盖区域。第一阶段的薄云处理有利于后面的STGAN捕捉到更多的地面信息。实验结果表明,与传统的去云方法和深度学习Pix2Pix等算法相比较,该算法无论在视觉效果上,还是峰值信噪比(PSNR)与结构相似性(SSIM)等客观质量评价指标上,均有显著的提升,有利于光学遥感图像的进一步利用。
Cloud occlusion often occurs in optical remote sensing images, and cloud occlusion may reduce or even completely occlude some ground cover information in the images, limiting ground observation, change detection or land cover classification. Cloud removal is an important task that urgently needs to be solved. Thin clouds and thick clouds usually coexist in optical remote sensing images, and the single-frame remote sensing image cloud removal algorithm is only suitable for solving the problem of thin cloud occlusion. Therefore, how to use multi-temporal remote sensing images of the same area at different times to remove clouds has become is a hot issue. In order to make full use of the images in the same location without cloud time period to replace the cloud-occluded images to restore the ground area occluded by clouds, a two-stage cloud removal algorithm for multi-temporal remote sensing images based on U-Net and spatiotemporal generative network (STGAN) is proposed. The first stage is cloud segmentation, which directly uses the U-Net model to extract clouds and remove thin clouds for each frame of the multi-temporal cloudy satellite images. The second stage is image restoration, which directly uses STGAN to remove thick clouds, and input the 7 frames of ground images after removing thin clouds into the STGAN model to obtain a single, detail-rich cloud-free ground image. The generative model of STGAN adopts an improved multi-input U-Net to recover the corresponding irregularities thick cloud cover area by extracting key features from 7 frames of images at the same location at a time. The thin cloud processing in the first stage is beneficial to the subsequent STGAN to capture more ground information. The advantage of the proposed algorithm is that it can not only solve the problem that U-Net cannot handle cloud occlusion in thick cloud areas, but also capture more ground information than directly using STGAN for cloud removal, and the cloud removal effect is better. The experimental results on our dataset show that both in terms of subjective visual effects and objective quantitive evaluation indicators such as peak signal-to-noise ratio (PSNR) and structural similarity (SSIM), only using the first-stage U-Net model and only using the second-stage STGAN model for cloud removal are inferior to the two-stage cloud removal algorithm proposed in this paper, which fully verifies the effectiveness of the cloud removal algorithm in this paper. Compared with traditional cloud removal methods such as RPCA, TRPCA and deep learning algorithms such as Pix2Pix, the proposed algorithm is superior to the comparison algorithm and has a significant improvement, which fully verifies the advancement of the cloud removal algorithm in this paper. The proposed algorithm makes full use of the spatiotemporal information of multi-temporal cloudy satellite images of the same area at different times, and has good cloud removal performance, which is conducive to the further utilization of optical remote sensing images. Although the proposed algorithm has achieved a relatively good cloud removal effect, it also has certain limitations. When facing the cloud image sequence with a large area covered by thick clouds, the cloud removal effect of the algorithm is not ideal. In the follow-up research, the spatio-temporal features of image sequence frames will be explored to better reconstruct the large area covered by thick clouds.