下载中心
优秀审稿专家
优秀论文
相关链接
摘要
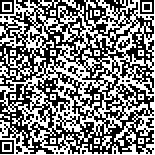
PM2.5作为指示环境质量的重要因子之一,不仅影响着灰霾天气的发生,还与公众健康息息相关,近年来受到广泛的关注。尽管PM2.5地面观测站点在不断地扩张,其覆盖范围依旧有限,难以反映全域PM2.5浓度的时空异质性。本研究运用卫星遥感气溶胶光学厚度数据,辅助因子除常规的气象因子等以外,还加入了针对中国人民生产生活习惯的农历日因子,提出一种耦合注意力机制的深度神经网络模型,对长三角区域2015年—2020年PM2.5浓度进行了逐日的高精度估算。模型交叉验证结果显示决定系数R2高达0.85,斜率0.86,与地面站点观测值有较高的一致性,优于多元线性回归和随机森林模型。长三角区域PM2.5浓度时空特征分析结果表明,PM2.5浓度在空间上呈现北高南低的趋势;季节特征以冬季浓度最高,夏季浓度最低,春秋过渡。此外,长三角区域2015年—2020年整体PM2.5浓度呈下降趋势,其中以上海市最为明显,下降速率为3.30 μg/(m3
PM2.5, as the primary indicator of environmental quality, not only affects the occurrence of haze but also is closely related to public health and has raised great attention recently. Although PM2.5 ground monitoring stations are expanding, they are still on the sparse side to identify the spatiotemporal heterogeneity of PM2.5 concentrations. With the development of remote sensing technology, satellite-based Aerosol Optical Depth (AOD) data provide an effective way to estimate large-scale PM2.5 concentrations. This study aims to develop a novel deep neural network model for estimating PM2.5 concentrations in the Yangtze River Delta (YRD).In addition to satellite remote sensing AOD data, meteorological factors, digital elevation model data, normalized different vegetation index data, and the lunar calendar day representing Chinese production and living habits were integrated into the proposed attention-based Self-Adaptive Deep Neural Network (SADNN) in this study to estimate PM2.5 concentrations in the YRD region from 2015 to 2020. Five-fold cross-validation was executed to evaluate the estimation accuracy of the SADNN. The multiple linear regression and random forest models were applied to compare with the SADNN.The cross-validation results showed the proposed SADNN model had a high coefficient of determination value of 0.85 and a slope of 0.86, which were highly consistent with ground-level observations. The results also showed better performance than those of multiple linear regression and random forest models. The results for the spring festival in 2016 demonstrated the effectiveness of integrating the lunar calendar day and attention module into the model. The spatiotemporal patterns of PM2.5 in the YRD were as follows: PM2.5 concentrations were high in the north and low in the south, and the coastal and mountainous areas were better than inland and plain areas, respectively. On seasonal scales, winter was the most polluted season, while summer was the best. The overall PM2.5 concentration in the YRD showed a decreasing trend from 2015 to 2020, especially in Shanghai Municipality, with the decreasing speed of 3.30 μg/(m3