下载中心
优秀审稿专家
优秀论文
相关链接
摘要
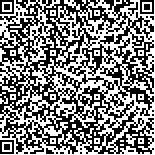
多光谱数据的降维处理对基于深度学习的单木树冠检测研究有重要意义,如何使用合适的降维方法以提高单木检测的精度却少有研究讨论。本文使用无人机搭载多光谱相机进行航拍作业,采集研究区内银杏树种多光谱影像。将原始多光谱影像通过特征波段选择、特征提取、波段组合的方法生成5种不同的数据集用于训练3种经典的深度学习网络FPN-Faster-R-CNN,YOLOv3,Faster R-CNN。其中由波段组合方法得到的近红外、红色、绿色波段组合在不同类型的目标检测网络中都有最好的检测结果,其中FPN-Faster-R-CNN网络对银杏树冠的检测精度最高为88.4%,由OIF指标得到的蓝色、红色、近红外波段组合信息量最高,但在所有网络中的平均检测精度最低,仅为79.3%。实验结果表明:在不同波段降维方法中,若降维后的影像中目标物体的色彩与背景差异较明显,且轮廓清晰,则深度学习网络对树冠的检测可获得较好的结果。而影像自身的信息量则对深度学习网络的树冠检测能力的提升作用有限。本研究中针对多光谱影像的降维方法分析,为基于深度学习的单木树冠检测研究提供了重要的实验参考。
The dimensionality reduction processing of multispectral data is of considerable importance to deep learning-based single-tree crown detection research. However, how to use the appropriate dimensionality reduction method to improve the accuracy of single-tree detection is rarely discussed. In this work, an unmanned aerial vehicle equipped with a multispectral camera was used for aerial photography to collect multispectral images of ginkgo tree species in the research area. The original multispectral images were used to generate five different data sets through feature band selection, feature extraction, and band combination method for training three classical deep learning networks: FPN-Faster-R-CNN, YOLOv3, and Faster R-CNN. Based on the characteristics of the band selection method, the red, green, and near-infrared bands combined with different types of target detection in the network have the best results. The FPN-Faster-R-CNN network detection accuracy is up to 88.4% for ginkgo trees. The blue, red, and near-infrared band combination obtained by the OIF index has the highest amount of information but the lowest average network accuracy at 79.3%. Experimental results show the following. In the different dimensionality reduction methods, if the color and background of the target object in the image after dimensionality reduction are obviously different, and the contour is clear, the deep learning network can obtain better results in tree crown detection. However, the information content of the image itself has a limited effect on the ability of the deep learning network to detect tree crowns. In this study, the dimensionality reduction method of multispectral images is analyzed, providing an important experimental reference for the deep learning-based single-tree crown detection.