下载中心
优秀审稿专家
优秀论文
相关链接
首页 > , Vol. , Issue () : -
摘要
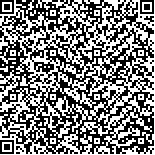
三维辐射传输模拟是研究定量遥感机理,提高反演精度的重要方法。现有研究能重建厘米级的植被场景,实现分米级的星载或者机载图像模拟。然而在稀疏植被区域,模拟图像和实际数据仍然存在一定偏差,一方面源于三维结构误差,另外一方面则是平均组分光谱的局限,难以反映地表反射率的随机异质性。为克服第二个问题,本文扩展RAPID模型,基于土壤端元和浓密植被端元光谱,增加了两个权重系数构成的纹理矩阵,实现地表反射光谱的精细模拟,并评估考虑异质性对图像模拟带来的改进效果。结果表明:(1)两个权重系数可以基于真实图像直接求解;(2)每个地表面元在赋值两个异质权重系数后,合成光谱与真实图像光谱曲线吻合度高(R2优于0.98, RMSE=0.016);(3)云南天峰山和甘肃大野口两个场景的模拟吻合度显著提升(R2分别提升0.096和0.041, RMSE分别下降0.015和0.01);(4)三波段RGB图像也有可能预测出NIR波段图像,纹理相近,但是光谱反射率数值上有一定的偏差(0.006~0.027)。本文提出的方法可以模拟更为精细的亚米级高分卫星图像,可为深度学习提供高质量的样本,为定量反演提供更准备的训练数据。
[Objective] It is an important method to use three-dimensional (3D) radiative transfer (RT) simulation to study the mechanism of quantitative remote sensing and improve the inversion accuracy. Computer graphics combined with laser scanning data or photogrammetry images can help produce realistic centimeter-level vegetation scenes, including leaves, branches, trunks and ground polygons. Current 3D RT models are able to utilize these kinds of reconstructed scenes to simulate decimeter-level spaceborne or airborne remote sensing images. However, in sparsely forest areas, there are still some deviations between the simulated and the actual images. It is somehow due to the reconstruction error of 3D structure of the trees or understorey; while the limitation of the average component spectrum of soil background plays another role, which is difficult to reflect the random heterogeneity of surface reflectance. [Method] In order to solve the second problem, this paper extends the 3D RAPID (Radiosity Applicable to Porous IndiviDual Objects for directional reflectance over complex vegetated scenes) model, adding two weight-coefficient texture matrices based on the end-member spectra of bare soil and dense vegetation, realizing the finer simulation of surface reflection spectrum. A multi-spectral or hyper-spectral image, or at least a RGB image containing the background texture information should be given as input. First, Normalized Difference Vegetation Index (NDVI) can be used to extract the pixel spectra of bare soil (NDVI~0.2) and dense vegetation (~maximum NDVI). Second, the two weight coefficients for each background pixel are fitted. Last, RAPID simulates the reflectance image using the texture matrices filled with the weight coefficients. Using real images from Tianfeng Mountain of Yunnan Province and Dayekou of Gansu Province, the improvement of image quality by considering heterogeneity on image simulation has been evaluated. The Tianfeng Mountain scene represents a sparse Yunnan pine (Pinus yunnanensi) forest with heterogeneous background (bare soil, shrub, grass, or dead wood) in the stand, using a hyperspectral image as input. Dayekou scene shows the Qinghai spruce (Picea carassifolia) forest segmented by a large grass patch, using a Quickbird image as input. [Results] The results show that: (1) the two weight coefficients can be solved directly from real images when the end-member of bare soil and dense vegetation are chosen properly; (2) If assigning two heterogeneous weight coefficients to each surface pixel, there is high coincidence between the synthetic spectrum and the spectral curve of the real image (R2 is better than 0.98, RMSE = 0.016); (3) The simulated image qualities are significantly improved in Tianfeng Mountain and Dayekou scenes (R2 increased by 0.096 and 0.041 respectively, and RMSE decreased by 0.015 and 0.01 respectively); (4) It is also possible to predict near infrared (NIR) band images from three-band RGB images. When using only RGB images as input, similar texture was reproduced, but there is a certain deviation in the NIR reflectance values (0.006 ~ 0.027). [Conclusion] The method proposed in this paper can simulate more precise sub-meter high resolution satellite images, which can provide high-quality samples for deep learning, as well as better training data for quantitative inversion.