下载中心
优秀审稿专家
优秀论文
相关链接
摘要
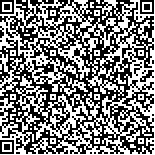
精确估算森林蓄积量是国家实现2060年前碳中和目标的迫切需求,而基于遥感的森林蓄积量定量反演是当前遥感应用领域面临的重要挑战和研究热点。光学遥感数据由于无法获取森林高度信息并存在信号饱和问题,反演森林蓄积量的精度较低,而机载Lidar数据能获取高度信息,但成本高、观测范围有限。本研究利用Sentinel-2多光谱、资源三号立体成像和机载Lidar数据,定量评价多源遥感数据在北亚热带估测森林蓄积量的能力,并探索小样本情况下贝叶斯模型在森林蓄积量估算中的优势,旨在解决单一数据集估测森林蓄积量精度低和样本数量不足的问题。研究结果表明,协同Sentinel-2和林分冠层高度模型(CHM)数据的贝叶斯分层建模能够显著提高森林蓄积量估测精度,其相对均方根误差(rRMSE)为27.6%,其估测误差比Sentinel-2数据减少了13.6 m3/ha,比单独CHM数据减少了7.4 m3/ha。多源数据可以有效改善低值高估和高值低估问题,相比单一数据集,多源数据在低值区估测误差减少了约1/4,在高值区减少了约1/3。分层贝叶斯模型有效缓解了因精细分层对样本数量的需求,减少了森林类型和地形差异对森林蓄积量估测精度的影响。为亚热带森林类型和地形复杂的地区,开展森林蓄积量估测制图提供关键技术手段。
Accurate estimation of Forest Growing Stock Volume (FGSV) is needed to achieve the goal of carbon neutral. Quantitative inversion of FGSV using remote sensing technologies is still a research challenge. Optical remote sensing technology is one of the most important means for FGSV estimation, but cannot provide sufficiently accurate estimates due to lack of canopy structure features and data saturation problem. Although airborne Lidar can overcome the shortcoming of optical sensor data, its high cost in data collection and limited observation area constrain its extensive application. This research employs integration of Sentinel-2, ZY-3 stereo, and airborne Lidar data to explore the performance of FGSV estimation in north subtropical regions, and examines the advantages of using the hierarchical Bayesian approach to develop FGSV estimation models under the condition of small population of sample plots. The objective is to solve low modeling accuracy caused by the single sensor data and insufficient number of sample plots. The results indicate that the hierarchical Bayesian approach based on combination of Sentinel-2 and Canopy Height Model (CHM) data (subtraction of Lidar-derived digital elevation model data from ZY-3 stereo-derived digital surface model data) provides the best estimation results with relative Root Mean Square Error (rRMSE) of 27.6%. The Root Mean Square Error (RMSE) using this approach reduced by 13.6 m3/ha comparing with the RMSE based on Sentinel-2 data alone, and reduced by 7.4 m3/ha based on CHM data alone. The research shows that use of multi-source data can effectively improve the problems of overestimation when FGSV is small and of underestimation when FGSV is relatively high, that is, use of multi-source data can reduce the overestimation by one forth and the underestimation by one third comparing with use of single data source alone. Comparing with traditional modeling approaches such as linear regression and random forest, the hierarchical Bayesian approach can effectively reduce the requirement of number of samples due to use of stratification strategy and reduce the impacts of forest types and terrain differences on FGSV estimation accuracy. This research provides new insights of using integration of different data sources to develop FGSV estimation models to achieve accurate estimates, and provides key technology for FGSV mapping in subtropical regions.