下载中心
优秀审稿专家
优秀论文
相关链接
摘要
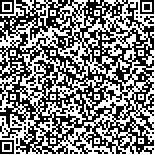
有机悬浮物OSM(Organic Suspended Matter)是湖泊有机碳库的重要组成成分,对研究湖泊生态环境和初级生产力具有重要意义。本研究以太湖、巢湖、滆湖、小兴凯湖、滇池、洪泽湖、呼伦湖和南漪湖8个内陆湖泊为研究区,发展了适合内陆水体的有机悬浮物浓度遥感估算方法。基于B6、B7波段的斜率和B10、B11波段的斜率,首先将水体分为两大类型,即以无机悬浮物占主导的A类水体和以有机悬浮物占主导的B类水体。在此基础上针对不同类型的水体分别建立OSM浓度的遥感估算模型,结果表明,A类水体OSM浓度估算可以利用波段比值B17/B3,B类水体OSM浓度估算可以利用波段比值B11/B10,RMSE为5.38 mg/L,MAPE为28.93%,与传统的非分类OSM估算经验方法相比,本文提出的方法,其估算的精度与分类前相比RMSE降低11.10 mg/L,MAPE降低41.66%。最后,将模型应用到2018-03-23太湖、2018-08-05太湖、2017-09-18巢湖和2017-12-21巢湖的OLCI影像上,结果表明OSM浓度空间分布特征与藻类水华和人类活动密切相关。
Organic Suspended Matter (OSM) is an important component of lake organic carbon pool, which is important to the study of lake ecological environment and primary productivity. At present, the research of OSM in water mainly focuses on its source composition, migration and transformation, and flux into the sea. Therefore, constructing a new method for estimating OSM in inland water is urgently needed. This method can obtain the spatial and temporal distribution characteristics of OSM concentration in the whole lake and even the whole region.In this study, the combined Taihu, Chaohu, Geui, Xiaoxingkai, Dianchi, Hongze, Hulun, and Nanyi lakes are selected as the research area, and a remote sensing method for estimating the concentration of organic suspended solids in inland water is developed. According to the slope of B6 and B7 bands and the slope of B10 and B11 bands, the water body is divided into two types, namely, the Type A water dominated by inorganic suspended matter and the Type B water dominated by OSM. On this basis, remote sensing estimation models of OSM concentration for different types of water bodies are established. The remote sensing estimation model of OSM concentration for Type A water is OSM=32.75·B17/B3-1.3537, and the remote sensing estimation model of OSM concentration for Type B water is: OSM=43.098+15.751·B10/Bl1. The band ratio B17/B3 can be used to estimate the OSM concentration of Type A water, and the band ratio B11/B10 can be used to estimate the OSM concentration of Type B water. The proposed method obtained RMSE of 5.38 mg/L and MAPE of 28.93%, which were decreased by 11.10 mg/L and 41.66%, respectively, compared with those of the traditional nonclassified OSM estimation empirical method.An empirical algorithm for retrieving OSM concentration in inland lakes is proposed on the basis of the band characteristics of Sentinel 3A-OLCI image. According to the spectral characteristics of inland water bodies, which are the slope of OLCI band B6 and B7 and the slope of band B10 and B11, the algorithm, which uses the strategy of first classification and then retrieval, can divide the water into two types: the water dominated by inorganic suspended matter and the water dominated by OSM. The OSM concentration of the water dominated by inorganic suspended matter can be estimated by using OLCI bands B3 and B17, while the OSM concentration of the water dominated by organic suspended solids can be estimated by using OLCI bands B10 and B11. Independent validation datasets show that the RMSE and MAPE estimated by this method are 5.38 mg/L and 28.93%, respectively. The estimation accuracy of this method is significantly improved compared with those of the existing retrieval algorithms for the concentration of OSM in inland water. This method has been successfully applied to obtain the temporal and spatial distribution characteristics of the OSM concentration in Taihu and Chaohu lakes in China. The method constructed in this study provides a new algorithm with higher accuracy for obtaining the OSM concentration of inland water at regional scale.The classification method of this study and the construction of the retrieval algorithm are based on several typical lakes on the basis of field sampling. However, the robustness of the algorithm still needs further examination, especially when it is applied to water with different biological optical characteristics from sampling lake. The performance of the algorithm also should be tested using a great number of field measured datasets. In future studies, the performance of the algorithm will be further tested by collecting more lake field data.