下载中心
优秀审稿专家
优秀论文
相关链接
摘要
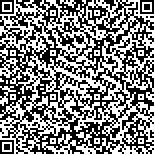
在浅水湖泊中,水生植物具有净化水质、抑制藻类、提供鱼类食物和栖息环境等生态功能,同时,其过度扩张也会加速湖泊淤浅和沼泽化、引起湖泊二次污染等环境负效应。实时动态地掌握湖泊水生植被类群和种群的空间分布及其面积、生物量等指标信息,对湖泊生态修复和评估、水生植被恢复和管理等具有重要现实意义。遥感技术的大面积、实时、动态等特点,为水生植被的现状监测、历史追溯、变化规律揭示等提供了有效手段。本文围绕“水生植被遥感”研究主题,开展了国内外文献调研,梳理了水生植被遥感监测的主要研究内容,并重点阐述了各内容的研究进展和方法等。最后,探讨了水生植被遥感存在的主要问题,并结合当前的遥感大数据发展,对未来水生植被遥感研究重点和发展趋势进行了展望。
In shallow lakes or reservior, aquatic vegetation plays an important role in purifying water, maintaining the balance of lake ecosystems, supporting socioeconomic functions and protecting lake ecological environment. However, an excessive amount of macrophytes, especially floating-leaved vegetation, can have some negative effects on lake ecology. For example, the addition of large amounts of plant material to the lake bottom can cause lake silting and accelerate lake swamping; the release of pollutants into the lake water when the plants die and decay can result in water pollution. Therefore, it is very important to map spatiotemporal distribution and their changes of aquatic vegetation and then to retrieve biochemical parameters such as coverage and biomass for ecological restoration and management of lakes. Remote sensing techniques have become powerful and effective tools for mapping aquatic vegetation types and their changes over a large area and a long period. In this paper, with the theme of aquatic vegetation remote sensing, we reviewed and summarized the major progresses and methods of remote sensing application in aquatic vegetation in shallow lakes by literature review. We found the research topics in aquatic vegetation remote sensing mainly included hyperspectral analyses, classification and mapping, parameter inversion, change detection, and so on. We also offered a literature statistical diagram of classification methods for mapping aquatic vegetation, and found decision tree was the most popular and machine learning was becoming more and more popular in all mapping methods. Finally, we discussed existing major challenges, potential solutions and future prospects in aquatic vegetation remote sensing, including developing a multi-parameter method for mapping different species of submerged vegetation, expanding the spatial-temporal scale of inversion models in parameters in application and making full use of the advantages of UAV (unmanned aerial vehicle) coupled with hyperspectral and multispectral sensors for mapping and parameter inversion in aquatic vegetation.