下载中心
优秀审稿专家
优秀论文
相关链接
摘要
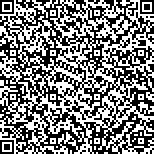
热红外遥感图像由于其特定的成像方式,包含目标特有的发射率及温度等特征。然而,热红外遥感图像较低的空间分辨率却限制了其广泛应用。随着遥感技术的发展,同一区域获得的多源遥感图像可以提供更为完备的目标信息,使得利用多源融合技术实现热红外图像空间分辨率增强与亚像素级特征提取成为可能。为此,本文提出了一种基于多分辨率自适应低秩表达与残差信息迁移的热红外图像空间超分辨算法,该算法通过可见光与热红外图像融合的方式实现热红外图像空间特性的自适应融合增强。本文算法优势主要体现在以下几个方面:(1)基于多分辨率的超像素分割,使用超像素块代替传统的方块作为低秩恢复单元,自适应地调整单元内空间特性以保持单元内地物类型的稳定并抑制结构性噪声;(2)通过构建导向线性滤波器,在保护热红外图像光谱信息的前提下,实现可见光图像精细空间特征向热红外图像的迁移;(3)在低分辨层建立增强热红外图像残差与可见光图像残差之间关联并迁移至高分辨层,在保证超分辨图像细节信息的前提下,实现热红外图像空间超分辨。为了验证算法的有效性,本文采用2014年IGARSS数据融合竞赛提供的可见光与热红外实验数据进行实验,并与融合竞赛中表现最为优异的监督图特征融合方法进行比较,并从温度反演精度以及分类精度两个方面评价超分辨效果。实验结果表明,本文提出的方法其噪声抑制效果、空间平滑效果、边缘锐化效果更为优异,超分辨热红外图像有着更为精细的空间信息,并且对于不同区域类型均能较好的保护热红外图像光谱信息。对于不同地物类型,融合超分辨图像有较高的亚像素温度反演精度以及更高的分类精度,其温度反演误差小于1 K,总体分类精度较原热红外图像提升20%以上。
Owing to the special imaging principle in the long-wave infrared region, thermal infrared remote sensing images contain the temperature and emissivity features of targets. However, the low spatial resolution of thermal infrared images limits its wide application. With the development of remote sensing technology, multisource remote sensing images in the same region can provide complete information of a target for researchers. On the basis of the high spatial resolution of visible band images, thermal infrared image fusion enhancement and subpixel feature extraction have a high application value.Therefore, a new method named subpixel temperature retrieval of thermal infrared images based on multiresolution superpixel low-rank representation and residual correlation is proposed in this paper. The method achieves two goals by fusing visible and thermal infrared images in a super-resolution way: (1) enhancement of spatial characteristics for thermal infrared images based on adaptive fusion and (2) estimation of subpixel temperature and super resolution for thermal infrared images.The main processing and advantages of the algorithm are listed as follows: (1) For superpixel segmentation and low-rank restoration at multiresolution, superpixel blocks, instead of traditional blocks, are used as low-rank restoration units to enhance the stability of species in each unit and suppress structural noise. (2) Through constructing a guided linear filter, the high-spatial-resolution feature of the visible image can be transferred to the thermal infrared image while keeping the spectral information of the thermal infrared image unchanged. (3) For the estimation of subpixel temperature and super resolution of thermal infrared images, the correlation between the residuals of VIS and fusion images is established at the low-resolution layer and applied to the high-resolution layer to preserve image details.To validate the effectiveness of the proposed method, the visible and thermal infrared data in the 2014 IGARSS data fusion contest are used for experiments. The algorithm is evaluated in three aspects: (1) the improvement of spatial characteristics for thermal infrared images through adaptive low-rank representation, such as noise suppression, intraclass smoothing, and edge enhancement; (2) the spectral information protection of thermal infrared images in homogeneous and heterogenous regions; (3) the super-resolution effect and the accuracy of subpixel temperature retrieval. Compared with the traditional supervised graph-based feature fusion method, the proposed method has the best edge-sharpening, noise suppression, and spatial smoothing effects. It can protect the spectral information of thermal infrared images for different region types. The super-resolution image obtained by the proposed algorithm achieves high-temperature retrieval accuracy, and the overall root-mean-square error is less than 1 K. The average classification accuracy is improved by more than 20%.