下载中心
优秀审稿专家
优秀论文
相关链接
摘要
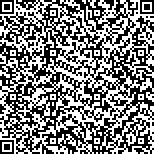
玉米作为中国重要粮食作物,品种众多,易出现错分现象,影响农业安全和粮食生产。针对传统基于卷积神经网络CNN(Convolutional Neural Network)的高光谱图像作物品种识别模型所需建模样本数量巨大的问题,提出基于高光谱像素级信息和CNN的玉米种子品种识别模型。首先,获取不同品种玉米种子在400—1000 nm范围内的高光谱图像,提取样本全部像素的203维光谱信息,利用主成分分析PCA(Principal Component Analysis)算法将光谱维度降至8维。在实验中,样本的像素级光谱信息(即:样本的全部像素的光谱信息)除应用于CNN模型外,也应用于支持向量机(SVM)和K近邻分类(KNN)模型中,结果表明:在相同模型中,基于像素级光谱信息比基于米粒级光谱信息(即:每粒样本所有像素光谱信息的平均值)识别效果好;在相同情况下,CNN模型比SVM和KNN模型的识别效果好;基于像素级光谱信息和CNN的品种识别模型识别效果最稳定,依据像素级分类结果采用多数投票策略对玉米种子样本进行识别,样本识别精度高达100%(注:100%为建模集样本与测试集样本数量为0.27和0.32时的识别精度,随着测试集样本数量的增加,该识别精度将有所降低)。最后,使用t分布随机邻域嵌入(t-SNE)算法实现CNN输出特征值的可视化,验证了基于高光谱像素级信息和CNN的品种识别模型的有效性。在建模样本极少的情况下,实现了玉米种子品种的无损、高效识别,为精准农业提供了理论基础。
As an important grain crop in China, maize has many varieties and is prone to misclassification, affecting agricultural security and food production. With the development of hyperspectral imaging and deep learning technology, identifying crop varieties using a combination of both is possible. A Convolutional Neural Network (CNN), the most representative algorithm of deep learning technology to deal with the image classification task, needs a large number of training samples in the model training process. However, obtaining a large number of hyperspectral images of maize seed samples is difficult and time consuming. Aiming at the problem of the large number of modeling samples required for the traditional method based on CNN for crop identification in hyperspectral images, a variety identification model for maize seeds based on hyperspectral pixel-level spectral information and CNN is proposed. First, hyperspectral images of different varieties of maize seeds in the range of 400—1000 nm are obtained, and 203-dimensional spectral information of all the pixels of samples is extracted. Nevertheless, the enormous amount of spectral information creates the problem of dimensional disaster and greatly increases the computational cost. Second, to reduce the dimensionality of the sample spectral information, the principal component analysis algorithm is used to reduce the spectral dimension to eight dimensions, which effectively shortens the operation time. Third, the pixel-level spectral information of the sample (i.e., the spectral information of all the pixels of the sample) is applied to the Support Vector Machine (SVM) and K-Nearest Neighbor (KNN) classification models, in addition to the CNN model. The experimental results demonstrate that for the CNN, SVM, and KNN recognition algorithms, the pixel-level spectral information models show a more stable and efficient recognition effect than the seed-level one (i.e., the average of all pixel spectral information of each sample). The seed-level information model does not fully utilize the sample pixel spectrum and spatial information, which needs a large number of modeling samples. When the number of samples used to build the classification model is the same, the CNN model has a significantly better recognition effect than the SVM and KNN models. In accordance with all the pixel-level classification results, a majority voting strategy is used to identify corn seed sample variety, and the sample recognition accuracy is up to 100% (100% refers to the identification accuracy when the numbers of samples in the modeling and test sets are 0.27 and 0.32, respectively. As the number of samples in the test set increases, the identification accuracy decreases). Lastly, the t-distribution random neighbor embedding algorithm is used to realize the visualization of output eigenvalues of CNN. The features of different maize seed varieties are clearly bounded in the visualization, which adequately verifies the validity of the species recognition model based on hyperspectral pixel-level information and CNN. In the rare case of modeling seed samples, nondestructive and efficient variety identification of maize seeds is realized, which will provide a theoretical basis for precision agriculture.