下载中心
优秀审稿专家
优秀论文
相关链接
摘要
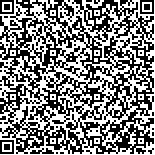
合成孔径雷达(SAR)因其对地观测全天候、全天时优势,成为多云多雨天气限制下洪水动态监测中不可或缺的数据来源之一。由于GEE(Google Earth Engine)云计算平台的兴起和短重访Sentinel-1数据的可获取性,洪水监测与灾害评估目前正面向动态化、广域化快速发展。顾及洪水淹没区土地覆盖变化的复杂性和发生时间的不确定性,基于时序Sentinel-1A卫星数据提出了针对大尺度范围、连续长期的汛情自动检测及动态监测方法。该方法首先,利用图像二值化分割时序SAR数据实现水体时空分布粗制图,逐像素计算时间序列中被识别为水体候选点的频率。然后,利用Sentinel-2光学影像对精度较粗的初期SAR水体提取结果进行校正,得到精细的水体分布图。最后,针对不同频率区间的淹没特点,采用差异化的时序异常检测策略识别淹没范围:对低频覆水区利用欧氏距离检测时序断点,以提取扰动强度大、淹没时间短的洪涝灾害区;对高频覆水区利用标准分数(Z-Score)检测时序断点,以提取季节性水体覆盖区。在GEE平台上利用该方法,实现了2020-05—10长江中下游地区全域洪水淹没范围时空信息的自动、快速、有效监测,揭示了不同区域汛情发展模式的差异性。本文提出的洪水快速监测方法对大尺度下的汛情动态监测、灾害定量评估和快速预警响应具有重要的现实意义。
Synthetic Aperture Radar (SAR) is an indispensable data source for the dynamic monitoring of flood events due to the capacity for all-weather and all-time sensing. At present, flooding mapping and damaging assessment are developed rapidly towards dynamic monitoring and large scale, owing to the emergence of cloud computing platforms, such as Google Earth Engine (GEE) and accessibility of short-revisit Sentinel-1A radar data. A novel method is proposed for detecting flood events and continuous monitoring of the inundated areas at a large scale based on the time-series processing and analysis from Sentinel-1A images, considering the complexity of land cover changes within the flooded areas and the temporal uncertainty of flood events. First, the binary segmentation threshold for the water extraction was determined by the maximum interclass variance algorithm, and accordingly, the coarse maps of spatiotemporal distribution of water body were generated from Sentinel-1A time series. Second, the frequency of candidate water pixels was computed through these coarse maps. The initial fine water map in the temporal sequence was refined by the water map retrieved by the NDWI from the Sentinel-2 optical image. In view of the distinctive inundation characteristics of different frequency areas, a differentiated sequential anomaly detection strategy was proposed to identify the flooded areas and the seasonal water areas. The change feature maps of backscattering coefficient were established by the Sentinel-1A time series. Euclidean distance was used to detect the sequential breakpoint in low-frequency water-covered area, which is regarded as the flooding disaster area with high disturbance intensity and short flooding time. Temporal Z-score was introduced to detect the breakpoint in high-frequency water-covered area, which is considered the continuous inundating area.Result We automatically, rapidly, and effectively detected the flood events in the middle and lower reaches of the Yangtze River Region between May and October in 2020 and monitored the inundated area change during flooding, using the proposed method deployed on the GEE. The results exhibited that the flooding areas, such as the middle reaches of the Huaihe River, the lower reaches of the Xinyi River, and the Chaohu Lake Basin, and displayed the max extents of seasonal water, such as Poyang Lake, Dongting Lake, and Shijiu Lake during extreme weather with serious precipitation. The storage, diversion, and release of the flood water in the Yangtze River Plain demonstrated a lower loss manner due to the function of the free Yangtze-linking lakes, such as Poyang Lake and Dongting Lake. In the Huaihe River Basin, the extreme rainy weather is more likely to result in flood disasters and causes public damage. The flood processing in the rainy season was described in a spatial fashion, and the regional differentiation of inundation patterns was revealed through the spatiotemporal information of the middle and lower reaches of the Yangtze River Region. The proposed rapid and robust flood monitoring method is greatly practical and important to the dynamic monitoring of flood situation, quantitative assessment of flood disaster, and rapid early warning response.