下载中心
优秀审稿专家
优秀论文
相关链接
摘要
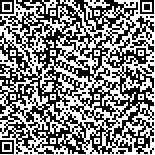
陆表温度是全球气候观测系统的基本变量。由于地表目标多数为具有三维结构、组成成分复杂、温度分布不均一的混合像元,因此相较于平均温度,组分温度具有更明确的物理意义和应用价值,对地—气相互作用过程和水分循环的定量分析研究具有重要意义。本研究针对Sentinel-3 SLSTR数据,基于CBT-P模型和CE-P模型构建了植被—土壤组分温度反演框架,并分析了劈窗算法和LAI对反演误差的影响。使用小汤山、漯河、塞罕坝3地实测数据进行结果验证,结果表明,5个验证点的植被组分温度反演结果的绝对偏差在0.1—1.6 K之间,平均绝对偏差为1.1 K,土壤组分温度反演结果的绝对偏差在0.5—1.4 K之间,平均绝对偏差为0.8 K,在中纬度的稀疏连续植被和垄行植被冠层中取得了较高的精度,初步证明了本研究提出的Sentinel-3 SLSTR双通道双角度地表组分温度反演算法的可行性。
Land surface temperature is the basic variable of the global climate observation system. Since most of the surface targets are mixed pixels with three-dimensional structure, complex composition, and uneven temperature distribution, compared with the average temperature, the component temperature has a clearer physical meaning and application value which is of great significance for the earth-atmosphere interaction process and quantitative analysis of water cycle. Vegetation and soil are two ground objects with completely different thermal properties in the vegetation-soil system. Obtaining accurate vegetation/soil component temperature is considered a prerequisite for improving the surface energy balance model. To develop component temperature inversion technology, this study proposes a new component temperature inversion algorithm based on Sentinel-3 SLSTR data.Based on the CBT-P model and the CE-P model, this study constructed a vegetation-soil component temperature inversion framework, and analyzed the impact of the split window algorithm and LAI on the inversion error. 946 clear sky atmospheric profiles were selected and the split window algorithm was used to obtain the surface brightness temperature. Then, LAI retrieved from the VNIR bands is used together with the measured component emissivity to be presented into the CE-P model to calculate the emissivity matrix. Finally, build an inversion framework based on the CBT-P model, and input the surface radiant brightness temperature and emissivity matrix to invert the component temperature.The results are verified using the measured data from Xiaotangshan, Luohe and Saihanba that show a high inversion accuracy can be achieved. The site vegetation types in these three places are mainly wheat and grassland, which belong to sparse vegetation and ridge crops respectively. At 5 sites, the vegetation component temperature retrieval error is between 0.1—1.6 K, and the average absolute error is 1.1 K. The soil component temperature retrieval error is between 0.5—1.4 K, and the average absolute error is 0.8 K. The single-factor error analysis results show that when the CWV is low, the split-window algorithm proposed in this study can bring about 1 K error to the vegetation component temperature inversion and bring no higher than 2.5 K error to the soil component temperature inversion which has a highly reliable inversion result. However, the error increases significantly in areas with high CWV, and the scope of application is limited. In the LAI single factor error analysis, when the LAI is large, the LAI error has a greater impact on the soil component temperature. When the LAI is greater than 4, 20% of the LAI error will cause the soil temperature inversion error to be greater than 3 K; when the LAI is small, the LAI error has a greater impact on the vegetation temperature. When the LAI is less than 1, the vegetation temperature retrieval error caused by LAI is close to 1 K.The result proves the feasibility of the Sentinel-3 SLSTR dual-channel dual-angle land surface component temperature inversion algorithm proposed in this study in the typical sparse vegetation and the row crops. However, this algorithm also has some shortcomings. The framework still has significant defects in areas with high CWV, and it still needs further verification in dense and continuous vegetation areas. In addition, this algorithm is based on the assumption of binary temperature and cannot handle the situation where the surface brightness temperature of the sensor 0° image is less than the 55° image, which is not uncommon in the actual inversion process. The component temperature inversion framework proposed in this study has the potential for integrated retrieval of atmospheric parameters and surface component temperature, which is worthy of further improvement.