下载中心
优秀审稿专家
优秀论文
相关链接
摘要
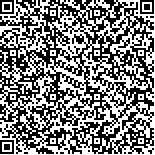
高光谱数据具有丰富的光谱特征,但是其空间分辨率相对较低。一些遥感数据具有与高光谱数据互补的优势,例如提供更精细的空间信息的高空间分辨率数据和具有高度信息的激光雷达LiDAR(Light Detection and Ranging )数据。通过将高光谱数据与多源遥感数据进行融合,可以弥补高光谱数据空间分辨率相对较低,空间特征不够丰富的缺点。近年来,基于深度学习的方法已经在遥感数据分类研究中取得了一定的进展。然而,由于深度网络的特征提取过程是一个自主的过程,往往无法精确的获取最有利于遥感数据分类的特征;同时,深度学习方法具有复杂的网络结构和大量的参数,往往会在分类训练过程中造成参数拟合困难。以上这些因素会导致分类效果不佳。针对这些问题,本文提出了一种将卷积神经网络CNN(Convolutional Neural Network)和纹理特征相结合的多源遥感数据特征级融合分类框架。该方法共3个步骤,首先,对高光谱数据或多源遥感数据提取纹理特征;然后,构造CNN,分别将原始高光谱遥感数据、原始多源遥感数据和第一步中获得的纹理特征作为深度网络的输入进行深度特征提取;最后,将分别提取到的深度特征拼接,并利用Softmax分类器进行分类。为了验证本文提出方法的分类效果,本文在休斯顿和塞特福德矿地区公开数据集上进行实验,并将该分类框架与支持向量机分类方法、像素级融合分类方法和特征级融合分类方法进行对比。由此可以分析得出,本文提出的基于深度学习的融合分类方法可以获得较高的分类精度。
Hyperspectral images (HSIs) have abundant spectral characteristics. However, their spatial resolution is relatively low. Some remote sensing data have complementary advantages with HSIs, such as the LiDAR, which can provide elevation information, and the high spatial resolution data, which have precise spatial information. The combination of HSIs with the multi-source remote sensing data for fusion classification can make up the deficiency of tits relatively low spatial resolution. In recent years, deep learning-based methods have been investigated for hyperspectral remote sensing classification and have made breakthroughs. Meanwhile, the feature extraction process of the deep network is an independent process. Therefore, it may not obtain the most beneficial features for classification accurately and may influence the classification accuracy. At the same time, the techniques may not perform well when using limited training samples in HSIs because of massive parameters and complex network structure.Aiming at this problem, the frequently used traditional features of remote sensing data for classification are discussed in this paper. A new deep learning-based feature level data fusion classification framework that integrates traditional textural features into Convolutional Neural Network (CNN) approach (T-F-CNN) is proposed for the accurate fusion classification of HSIs and multi-source remote sensing data. The proposed method can be implemented in three steps. First, the traditional features are extracted from the HSIs or the multi-source remote sensing data. Second, CNN are built. The original HSIs, the original multi-source remote sensing data, and the traditional features, which are obtained in the first step, are inputted into the CNN of the deep feature extraction. Finally, the deep features obtained in the second step are concatenated in a concatenate layer of CNN, and SoftMax is used to generate classification maps at the end of the framework.Result The proposed classification scheme is tested on two data sets, namely, Houston and Thetford Mines Area data sets. The proposed T-F-CNN is compared with the pixel-level methods, such as Support Vector Machines (SVM) with the Radial Basis Function (RBF) (T-P-SVM), the CNN fusion method (P-CNN), and the CNN with traditional features (T-P-CNN); and the feature-level methods, such as CNN fusion method (F-CNN) and CNN method combined with original traditional features (T’-F-CNN). On both data sets, the proposed method shows a higher classification accuracy than other methods. Meanwhile, when the training samples reach a minimum number, the proposed method could provide the highest overall classification accuracy.The results obtained by the proposed method on the two real hyperspectral data sets demonstrate that the classification accuracy can be improved. Furthermore, the proposed T-F-CNN method outperforms some traditional deep learning methods and exhibits higher computing efficiency than a few advanced deep learning techniques.