下载中心
优秀审稿专家
优秀论文
相关链接
摘要
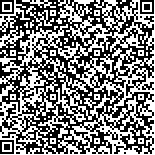
尾矿库是矿山企业选矿的必要设施,同时对周围环境也是一个重大的危险源。为研究流域范围内尾矿库的溃坝路径以及对矿区地表造成的风险,以赤城县为例,利用GF-1高分辨率遥感影像,基于遥感RS(Remote Sensing)及地理信息系统GIS(Geographic Information System)手段对尾矿库的流域风险进行了监测分析。首先,通过分析尾矿库在遥感影像上的纹理、色调、形状以及大小等特征,制作了用于目标检测的尾矿库样本集,然后,在原始SSD目标检测网络基础上添加了反卷积模块和连接模块构建多尺度融合目标检测算法MSF_SSD,在目标检测结果基础上使用PSPnet算法实现尾矿库结构分割,得到了尾矿库内部结构—坝体以及库区,运用RS与GIS技术对尾矿库的上游汇水面以及事故可能径流进行提取,进而基于Arc Hydro模型模拟尾矿库的溃坝路径。最后,通过构建溃坝路径的缓冲区,得到尾矿库发生溃坝所造成的地物影响范围及面积。研究结果表明:赤城县尾矿库的溃坝路径总体是从西向东,从北向南,受溃坝影响的地物总面积达到480 km2。其中,林地176.52 km2,耕地175.52 km2,城市建设用地43.74 km2,农村建设用地2.47 km2,水体17.72 km2,草地和牧场分别为3.60 km2、1.22 km2。研究成果可用于分析尾矿库溃坝造成的地物损失以及影响范围面积等信息,提升尾矿库的风险管理水平及应急响应能力,为有关部门制定决策提供理论依据。
Tailings reservoir is a necessary facility for mining activity, and it also causes danger to surrounding environment. . Watershed risk of the tailings reservoir in Chicheng was monitored and analyzed using GF-1 high-resolution remotely sensed data with the help of multiscale fusion and deep learning method, as well as the support of Remote Sensing (RS) and Geographic Information System (GIS) technology for a comprehensive and detailed identification and extraction of the risk information of the tailings reservoir and to study the dam-break path of the tailings reservoir in watershed and the risk to land surface over mining area.In this research, a sample set library for target detection was constructed by analyzing texture, hue, shape, and size of the tailings reservoir on the remotely sensed data. Subsequently, an improved multi-scale fusion algorithm (e.g., Multi_Scale Feature Map_SSD (MSF_SSD)) was constructed by adding a deconvolution module and a connection module to the original single shot multiBox detector (SSD). Next, the Pyramid Scene Parsing network (PSPnet) algorithm was selected to achieve the structure of the tailings reservoir on the basis of the target detection results. The internal structure of the tailings reservoir was obtained. With the help of RS and GIS technology, the surface of upstream catchment and the possible danger runoff were extracted, and dam-break path of the tailings reservoir is simulated on the basis of the arc hydro model. Finally, the range area affected by the dam-break were obtained by constructing the buffer zone of the dam-break path.The research results shown that the dam-break path of the tailings reservoir in Chicheng is generally from west to east and from north to south, and the total area affected by the dam-break was 480 km2. The combination analysis with land use/ cover classification indicated that forest land was 176.52 km2, farm land was 175.52 km2, urban land was 43.74 km2, rural construction land was 2.47 km2, water body was 17.72 km2, grassland was 3.60 km2, and pasture was 1.22 km2.The sample library constructed using GF-1 remotely sensed data and Google Earth 16 level image can provide the basis for the automatic recognition of tailings reservoir with deep learning framework. With the help of improved MSF_SSD and PSPnet algorithm, the semantic segmentation accuracy of the test area for pixel accuracy, mean IoU, F1 score, and mean F1 score is 0.98, 0.97, 0.99, 0.98, respectively. A comprehensive analysis of tailings reservoir dam-break range and possible damage to land surface types are performed with the help of hydrological analysis method and random forest classification results. Outcomes of this research can be used to analyze the impact area caused by dam-break, promote the capabilities of risk management and emergency response of tailings reservoir, and provide fundamental theories for decisions making in relevant departments.