下载中心
优秀审稿专家
优秀论文
相关链接
摘要
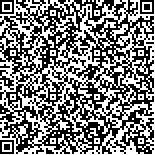
条件随机场模型由于其较强的上下文信息建模能力,被广泛应用于建筑物提取任务中。然而,面对高分辨率遥感影像丰富的地物信息,基于条件随机场的提取方法存在建筑物边界模糊的问题。本文提出了一种全局局部细节感知条件随机场框架,该框架提出全局局部一体化D-LinkNet,在有效利用多尺度建筑物信息的同时保留局部结构信息,解决了传统条件随机场一元势能丢失边界信息的问题。同时,该框架融合分割先验以缓解建筑物类内光谱差异较大的影响,利用更大尺度的上下文信息来精确提取建筑物,并引入局部类别标记代价从而保持细节信息以获取清晰的建筑物边界。实验结果表明,该框架在WHU卫星和航空数据集上的精度评价指标均优于其他对比方法,其IoU分别达89.82%和91.72%,对于复杂场景下的建筑物信息能够获得较好的提取效果。
Obtaining building distribution maps accurately and quickly has very important research value. For instance, it can help governments and non-governmental organizations plan urban infrastructure construction and assist disaster relief work. The Conditional Random Field (CRF) is widely used in building extraction tasks because of its flexible context information modeling and detail extraction capabilities. However, problems, such as blurry building boundaries, still exist when the CRF is used to extract buildings from high spatial resolution remote sensing images.This study proposed a Global-Local-Aware conditional random fields framework for building extraction. Global-Local-Aware D-LinkNet (GLD-LinkNet) is proposed to solve the boundary blur problem of unary potential in this framework. GLD-LinkNet makes up for the loss of local structural information by D-LinkNet while using multi-scale building information effectively. In addition, the segmentation priors are fused, and the label cost is introduced. The pairwise potential reflects the linear combination of the spatial relationship of neighboring pixels and the cost of the local class label. It can maintain the detailed information inside the buildings effectively. In addition, to solve the problem of spectral similarity between buildings and noise, a larger range of context information is used to fuse segmentation prior to the extraction of buildings. The framework can eliminate the influence of image noise and spectral diversity within class. Moreover, it can also keep the detailed information of ground objects and determine the clear boundaries of buildings.Experiments were carried out on the aerial building dataset and the satellite building dataset of the WHU building datasets. Experimental results demonstrate that the proposed dilated and segmented conditional random field framework is superior to the state-of-the art methods in terms of accuracy and IOU. The model proposed in this study, which performs well for building extraction of complex scenes, can maintain the detailed information of buildings effectively. In addition, it removes the small building blocks mistakenly extracted by D-LinkNet, and the problem of blurred building boundaries has also been improved effectively.The use of the global and local integrated D-LinkNet to model the unary potential of CRF can realize the effective combination of building features of different scales. It makes the structure of the obtained buildings more complete. Furthermore, by adding a segmentation prior to the construction of pairwise potential, a building classification map with a clean background can be obtained. The introduction of the local class label cost term also meets the high requirements of the building extraction task for the extraction of building detail information and can capture detailed information that is difficult to identify on the network. The proposed model was tested on aerial and satellite datasets, and the IoU indicators on the two datasets reach 91.72% and 89.82%, respectively. These values imply that the framework can adapt to aerial and satellite datasets.In the future, we will further study the application of large-scale high-resolution remote sensing images in building extraction and try to combine multi-source geographic information data to extract more complete building information.