下载中心
优秀审稿专家
优秀论文
相关链接
摘要
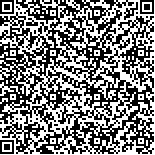
针对基于单系统单卫星GNSS-MR(GNSS Multipath Reflectometry)土壤湿度反演的可靠性不高、实际可操作性不强和最小二乘估计不具鲁棒性的缺点,为获取更优的延迟相位估值,并改善GNSS-MR土壤湿度反演的可靠性和实际可操作性,同时简化繁杂的选星过程,提出了一种基于抗差估计的多系统多卫星组合GNSS-MR土壤湿度反演算法。该算法首先顾及多径环境的差异性、多径误差的周期特性等进行信噪比SNR(Signal to Noise Ratio)观测值的筛选,然后采用基于IGGIII(Weight Function III Developed by Institute of Geodesy and Geophysics)权函数的抗差估计解求延迟相位,进而获得表征土壤湿度变化趋势的延迟相位组合。实验结果表明,相较于未采用抗差估计的多系统多卫星组合(方案1)和单卫星组合(方案3),得益于抗差估计良好的鲁棒性,基于抗差估计的多系统多卫星组合(方案2)和单卫星组合(方案4)获得了较高的建模精度,所得延迟相位与实测土壤湿度间的相关系数分别为0.97和0.95、土壤湿度拟合残差的均方根误差分别为0.010和0.012;同时,方案2和方案4还取得了较高的土壤湿度预报精度,土壤湿度预测值与土壤湿度实测值间的相关系数分别为0.92和0.91、土壤湿度预报残差的均方根误差分别为0.016和0.023;此外,相比于方案4,方案2在采用抗差估计解求延迟相位的基础上,采用多系统多卫星组合进一步提升了延迟相位的估值精度,从而不仅避免了复杂的选星过程,而且还获得了更好的建模效果和更高的土壤湿度预报精度。
关键词:
多系统多卫星GNSS-MR 土壤湿度反演 信噪比 延迟相位 抗差估计In order to obtain better phase delay estimation, improve the reliability and the practical operability of GNSS-MR(GNSS Multipath Reflectometry) soil moisture inversion, and also to simplify the complex process of the satellite selection, a multi-system multi-satellite GNSS-MR soil moisture inversion algorithm based on the robust estimation was proposed in view of the poor reliability and operability of the single system single satellite GNSS-MR soil moisture inversion and the least squares estimation of no robustness. In this algorithm, the spatial difference of multipath environment and the periodic characteristics of multipath were taken into account to screen the SNR (Signal to Noise Ratio) observations. Then, the phase delay combination representing the change trend of soil moisture was obtained by using the robust estimation based on IGGIII (Weight Function III Developed by Institute of Geodesy and Geophysics) weight function. Compared with multi-system multi- satellite combination (scheme 1) and the single-satellite combination (scheme 3), the experimental results showed that the multi-system multi-satellite combination (scheme 2) and the single-satellite combination (scheme 4) based on the robust estimation achieved higher modeling accuracy, which were benefited from the positive performance of the robust estimation. The correlation coefficients between the estimated phase delays and the measured soil moisture were 0.97 and 0.95, respectively, and the root mean square error of the soil moisture fitting residual were 0.010 and 0.012, respectively. At the same time, scheme 2 and scheme 4 also achieved higher soil moisture prediction accuracy, with the correlation coefficient between the predicted soil moisture and the measured soil moisture being 0.92 and 0.91, respectively, and the root mean square error of the soil moisture forecast residuals being 0.016 and 0.023, respectively. In addition, compared with scheme 4, scheme 2 not only adopted the robust estimation, but also adopted the multi-system multi-satellite combination, which contributed to better modeling effect and higher modeling accuracy. Moreover, because it could avoid the complex process of the satellite selection, scheme 2 owned better performance in GNSS-MR soil moisture inversion.