下载中心
优秀审稿专家
优秀论文
相关链接
摘要
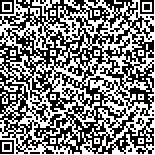
向量化的胶囊神经元和动态路由式的信息传递机制赋予了胶囊网络更强的特征表示能力。在遥感领域,基于胶囊网络的高光谱影像分类方法已经获得了较传统深度学习模型更为优异的分类结果。针对现有胶囊分类模型中存在的网络浅层、空谱联合信息利用不足等问题,本文利用卷积胶囊层、残差连接、三维卷积胶囊层构建了一种用于高光谱影像分类的新型深度胶囊网络。具体地,本文方法直接以高维数据立方体作为网络输入,并利用胶囊残差块逐层提取数据中的深层抽象特征。为了更加充分地利用影像中的空谱联合特征,在深层次的胶囊残差块中引入三维卷积胶囊层,以进一步提高分类精度。为了验证本文方法的有效性,选择University of Pavia、Indian Pines和Salinas等3个常用高光谱数据集和一个大规模机载高光谱数据集Chikusei进行实验。结果表明,与现有深度学习模型相比,本文方法能够获得更为优异的分类效果,在4个数据集上分别获得了99.43%、98.85%、97.14%和97.43%的总体分类精度。
Owing to vectorized capsule neurons and dynamic routing algorithm, capsule network possesses stronger feature representation capability than traditional convolutional neural networks. In the field of remote sensing, hyperspectral image (HSI) classification methods based on capsule network have obtained better classification results than traditional deep learning models. Aiming at the problems in the capsule classification models, such as shallow network layers and insufficient utilization of spatial–spectral information, this paper constructs a new deep capsule network for HSI classification. The designed network utilizes convolutional capsule layer, residual connection, and three dimensional convolutional capsule layer to further improve classification accuracy.The proposed method includes a convolutional layer, four capsule residual blocks, a class capsule layer, and a reconstruction network. First, the proposed method takes HSI data cubes as the input directly to retain the spatial–spectral details in the HSI. Then, the deep features in the input data are extracted layer by layer with the capsule residual blocks. Finally, the three dimensional convolutional capsule layer is introduced to make full use of the spatial–spectral information, so as to improve the classification accuracy.Three public HSI data sets including University of Pavia, Indian Pines and Salinas, as well as a large-scale hyperspectral data set Chikusei, are selected for the experiments. The results demonstrate that the proposed method outperforms the existing deep learning classification methods. Compared with the shallow capsule network model and the deep three dimensional convolutional network with residual structure, the proposed method improves the overall classification accuracy by 1%—3%, 2%—5%, 1%—2%, and 2%—4% on four different data sets. In addition, hyperparameters such as learning rate, capsule neurons dimension, network structure, and spatial neighborhood have been analyzed in detail. The effectiveness of three dimensional convolutional capsule layer has also been proven by conducting ablation studies.Compared with the existing HSI classification model based on capsule network, the proposed method has the following advantages. (1) The high-dimensional cubes are used for input data without any dimension reduction process, thus enabling the model to make full use of spatial–spectral details. (2) A deep network is constructed utilizing convolutional capsule layers and residual connection, thus allowing the model to extract more robust and abstract deep features. (3) The introduction of three dimensional convolutional capsule layer can make full use of spatial–spectral information in HSI to further improve the classification accuracy.