下载中心
优秀审稿专家
优秀论文
相关链接
摘要
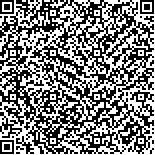
为了充分利用多光谱图像的光谱信息和全色图像的空间信息,本文提出一种基于深度递归残差网络的遥感图像空谱融合方法。方法将残差网络和递归网络相结合,利用残差网络学习低空间分辨率多光谱图像与高空间分辨率多光谱图像之间的残差,同时结合全局残差和局部残差,加快网络的收敛速度,解决深层次网络容易出现的梯度消失和梯度爆炸问题;利用递归神经网络在不增加权重参数、减轻过拟合的情况下通过提高网络层数来提高精度,得到更好的图像融合效果。为了验证本文方法的有效性,应用遥感图像进行模拟实验、真实实验和泛化实验,实验结果与传统方法和现有深度学习方法进行对比分析。主观视觉和客观定量评价表明,本文方法很好地改善了传统方法存在的光谱失真现象,并且较现有深度学习方法学习到更深层次丰富的图像特征,更好地保留了图像的空谱信息,同时泛化实验也说明本文的网络具有较好的泛化能力。
Pan-sharpening is a task in the field of remote sensing data fusion, in which multispectral (MS) images with rich spectral information but low spatial resolution and panchromatic (PAN) images with rich spatial details but only grey information are fused to yield images with high spatial and spectral resolution. Traditional Component Substitution (CS) methods replace a particular component of the MS image transformation with a PAN image, and then inversely transforms it to obtain the final fused image. The traditional MultiResolution Analysis (MRA) methods first extract spatial structures from the PAN image by using MRA transforms, and then the extracted spatial structure information is injected into the up-sampled MS images to obtain the fused image. The whole fusing process of the CS and MRA methods can be described as linear functions. However, the performance of such linear models are limited by their linearity, which often has spectral distortion. In recent years, many advanced nonlinear deep learning models have been proposed. However, those existing deep learning fusion models are relatively simple and pose difficultly in learn in-depth features. To overcome the shortcomings of the current models, we propose a deep recursive residual network that is specifically designed for the pan-sharpening task.Considering that the low-resolution input image and the high-resolution output image have high similarity, learning the relationship between input and output is highly redundant and difficult. If the sparse residual features between input and output are learned directly, then the network convergence can be significantly improved. Thus, the residual learning introduces the network structure, in which the introduced residuals include global residuals and local residuals. Such a structure is conducive to learning and not prone to overfitting. Moreover, the residual network can solve the problem of deep network gradient disappearance and gradient explosion well. Recursive network improves accuracy by increasing the number of network layers without increasing weight parameters. Specifically, as we use the residual network globally, recursive learning is introduced into residual learning by constructing recursive blocks structure, whereas multiple local residual units are stacked together in the recursive block. Through such an end-to-end network design, a better image fusion effect is obtained.Given that no ideal fusion result has been used as a label, we made a data set according to Wald’s protocol using the original MS as the ideal fused image, downsampling and then upsampling the MS as the MS of the network input, and the downsampled PAN as the PAN of the network input. To comprehensively analyze our experimental results, we performed a large number of simulation experiments and real experiments on the 4-band GaoFen-1 data and 8-band WorldView-2 data with abundant feature types. We then generalized them to 4-band GeoEye data and 8-band WorldView-3 data. Experimental results are compared with traditional methods and the existing deep learning methods. The subjective visual analysis and objective evaluation indicators show that the proposed method reduces the spectral distortion phenomenon of traditional methods and preserves the spectrum of an image better than the existing deep learning method does.The deep network designed in this paper has learned more in-depth and more luxurious image features and has achieved better fusion effects than existing methods. It uses a residual network to solve profound network gradient disappearance, gradient explosion, and degradation problems. In addition, the weight parameters are reduced by the design of the recurrent recursive block, and the network speed is improved. The generalization experiment shows that our network has a good generalization ability.