下载中心
优秀审稿专家
优秀论文
相关链接
摘要
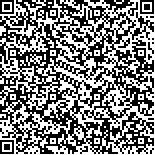
高分辨率遥感图像目标检测是计算机视觉的一个重要研究领域,在民用与军事领域具有重要的应用价值。目前,基于深度学习的自然图像目标检测有了突破性进展。但是,由于遥感图像具有目标尺度差异大且类间相似度高的特点,使得处理自然图像的目标检测算法直接应用于遥感图像时仍面临着一些挑战。针对上述挑战,本文提出一种多分辨率特征融合的遥感图像目标检测方法。首先,通过特征金字塔提取多尺度特征图并在其后嵌入多分辨率特征提取网络,促使网络学习目标在不同分辨率下的特征,缩小不同特征层之间的语义差距。其次,为实现多分辨特征的有效融合,本文采用自适应特征融合模块挖掘更具判别性的多分辨特征表达。最后,将自适应特征融合模块的输出特征的相邻层进行深度融合。在公开的遥感图像目标检测数据集DIOR和DOTA上评估了本文方法的有效性,相比采用特征金字塔结构的Faster R-CNN,本文方法的准确率(mAP)分别提高2.5%和2.2%。
In recent years, high-resolution remote sensing image object detection has attracted increasing interest and become an important research field of computer vision due to its wide applications in civil and military fields, such as environmental monitoring, urban planning, precision agriculture, and land mapping. The natural scene object detection frameworks based on deep learning have made a breakthrough progress. These algorithms have good detection performance on the open data sets of natural scenes. However, although these algorithms have greatly improved the accuracy and speed of remote sensing image object detection, they have not achieved the expected results. Given the large variations of object sizes and inter-class similarity, most of the conventional object detection algorithms designed for natural scene images still face some challenges when directly applied to remote sensing images. To address the above challenges, we propose an end-to-end multi-resolution feature fusion framework for object detection in remote sensing images, which can effectively improve the object detection accuracy. Specifically, we use a Feature Pyramid Network (FPN) to extract multi-scale feature maps. Then, a Multi-resolution Feature Extract (MFE) module, which can promote the network to learn the feature representations of the objects at different resolutions and narrow the semantic gap between different scales, is inserted into the feature layers of different scales. Next, to achieve an effective fusion of multi-resolution features, we use an Adaptive Feature Fusion (AFF) module to obtain more discriminative multi-resolution feature representations. Finally, we use a Dual-scale Feature Deep Fusion (DFDF) module to fuse two adjacent-scale features, which are the output of the adaptive feature fusion module. In the experiments, to demonstrate the effectiveness of each module of our proposed method, including the MFE, AFF, and DFDF modules, we first conducted extensive ablation studies on the large-scale remote sensing image data set DIOR, and the experimental results show that our proposed MFE, AFF, and DFDF modules could improve the average detection accuracy by 1.4%, 0.5%, and 1.3%, respectively, compared with the baseline method. Furthermore, we evaluate our method on two publicly available remote sensing image object detection data sets, namely, DIOR and DOTA, and obtain improvements of 2.5% and 2.2%, respectively, which are measured in terms of mAP comparison with Faster R-CNN with FPN. The detection results of the ablation studies and the comparison experiments indicate that our method can extract more discriminative and powerful feature representations than Faster R-CNN with FPN, which can significantly boost the detection accuracy. Moreover, our method works well for densely arranged and multi-scale objects. Although many improvements have been achieved in this work, some aspects still require improvement. For example, our method performs poorly in terms of detecting objects with big aspect-ratios, such as bridges, possibly because most anchor-based methods have difficulty ensuring a sufficiently high intersection over union rate with the ground-truth objects with big aspect-ratios. Our future work will focus on addressing these problems by exploring the advantages of anchor-free based methods.