下载中心
优秀审稿专家
优秀论文
相关链接
摘要
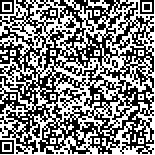
高分辨率遥感影像中的地物目标具有清晰的类别属性与空间关系语义。在人工智能技术支撑下,用计算机自动认知其空间关系具备了可行性。目前,遥感影像场景的语义理解主要依托图像描述任务(image caption),基于影像的全局特征生成描述语句。但是,这种粗粒度特征容易导致地物目标的类别属性在描述语句生成过程中被错误预测。事实上,以地物目标作为空间关系语义理解的基本单元,更符合人们认知地理空间的习惯。为得到更准确的描述语句,本文构建了基于地物目标的遥感影像语义理解数据集,并提出双LSTM驱动的地物目标空间关系语义理解方法。该方法用目标检测模型识别影像中的显著目标,将这些目标特征输入到语言模型,以缓解描述语句中类别被错误预测的问题。进而,为利用遥感影像场景信息,将影像全局特征与目标区域特征进行融合,并用双LSTM预测目标的注意力分布,提高描述语句生成质量。对比实验结果表明,该方法能生成更准确的图像描述。
Geo-objects in High-resolution Remote Sensing Images (HRSIs) have clear category attributes and rich semantic information. With the support of artificial intelligence technology, the spatial relationship can be automatically recognized by a computer. At present, the semantic understanding of HRSIs mainly relies on an image caption model to generate sentences based on the global features. However, coarse-grained features can easily cause the category attribute of the object to be mispredicted during the sentence generation process. In fact, taking the geo-object as the basic unit of semantic understanding is more in line with people’s habit of cognizing geographic space. To obtain more accurate sentences, this study constructs an Object-based Geo-spatial Relation Image Understanding Dataset (OGRIUD) and proposes a dual LSTM-driven semantic understanding method.The proposed dataset is based on the object, and the sentence description includes the category and location information of the ground object, which make up the deficiency of the target category and the location information in the semantic understanding of the current remote sensing field. The proposed method uses the object detection model to identify salient objects in the image and uses the object features as input in the language model to alleviate the problem of incorrectly predicted categories in the description. Furthermore, to use HRSI scene information, we fuse the global and regional features and use dual LSTM to predict the attention distribution of each geo-object.We compare the global feature-based approach with the object feature based approach proposed in this paper. Quantitative analysis results show that the proposed method exhibits increased exact matching accuracy, from 53.5% of the original to 62.33%. The visual analysis results show that the proposed method, and the generated spatial relation description statements are also more abundant.This method enables the language model to focus on objects with actual semantics, and the matching degree between the generated description statement and the remote sensing image content is also improved. The correspondence between the visual object and description improves the interpretability of remote sensing image understanding.