下载中心
优秀审稿专家
优秀论文
相关链接
摘要
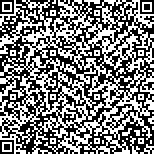
目前,高光谱遥感特征提取方法往往因受到噪声的干扰而导致降维效果欠佳。近年来,判别局部对齐DLA(Discriminative Locality Alignment)由于可以处理非线性分布样本、保留局部判别信息,同时避免矩阵奇异性问题,受到了很多学者的关注;但该方法无法有效估计和减少噪声对高光谱遥感影像的影响。针对以上问题,本文提出了最小噪声判别局部对齐MDLA(Minimum-noise Discriminative Locality Alignment)的线性特征提取方法和核最小噪声判别局部对齐KMDLA(Kernel Minimum-noise Discriminative Locality Alignment)的非线性特征提取方法。充分利用最小噪声分离MNF(Minimum Noise Fraction)的去噪能力,将MNF与DLA算法结合提出了MDLA方法,该方法首先利用MNF对高光谱遥感影像进行降维,减少图像中的噪声,然后再在子空间进行DLA变换得到最终的投影数据。为提高样本分布的非线性判别能力,基于KMNF与DLA算法将核方法引入MDLA,提出了KMDLA方法,该方法首先通过KMNF将原始空间的数据映射到新的特征空间,然后在特征空间中进行DLA变换得到最终的投影数据。实验部分首先利用3组高光谱遥感数据对提出算法的性能进行评价,并与相关特征提取算法进行了对比分析,最后分析了图像噪声对不同降维方法性能的影响。结果表明:提出的算法对高光谱遥感影像特征提取效果较好,且可有效减少噪声对影像的影响并提升其分类准确度。
Discriminative Locality Alignment (DLA) is a linear feature extraction algorithm, which has exhibited effectiveness in many fields. The algorithm can deal with the nonlinearity of samples distribution, preserve discriminative information over local patches, and avoid the problem of matrix singularity in calculation. Generally, the PCA step is recommended for reducing noise. However, PCA extracts noise images with low order components, and bands with a small variance do not necessarily indicate poor image quality. To effectively reduce the influence of noise on DLA and further improve the accuracy of DLA in the feature extraction of hyperspectral remote sensing images, a linear feature extraction algorithm of MDLA and a nonlinear feature extraction algorithm of KMDLA are proposed in this paper. The key idea of MDLA is as follows. First, MNF transforms data from the original space into a new subspace. In the process, the SNR is used to improve the order of components, and the noise in the image can be effectively reduced by sorting according to the image quality. Second, DLA is performed in the new subspace to obtain the result of feature extraction. KMDLA is the kernel method of MDLA. The main idea of KMNF is as follows. First, the samples are mapped to the high-dimensional feature space by the kernel function in which MNF is conducted. Finally, DLA is performed in the subspace spanned by KMNF, further enhancing the nonlinear discriminant ability of MDLA for the samples. Three sets of hyperspectral remote sensing images were selected as the study area. A detailed comparison of the proposed algorithms and five algorithms, namely, the PCA, MNF, LDA, CCPGE, and DLA algorithms, is provided in this paper. For a more intuitive comparison, experiments were conducted to classify the images of all bands. The support vector machine classifier was used to classify the results of feature extraction. Experimental results demonstrate that both MDLA and KMDLA outperform the five algorithms of comparison. Compared with DLA, the proposed algorithms exhibited respective improvements of 2.06% and 2.74% on the Purdue Campus data, 1.91% and 2.45% on the Indian Pines data, and 1.41% and 2.04% on the Salinas data. In terms of efficiency , MDLA and KMDLA consumed less operation time for classification than DLA when the experimental data was small. KMDLA consumed the most operation time when the experimental data was large, whereas MDLA always consumed minimal operation time. The effect of image quality on the performance of different dimensionality reduction methods is discussed based on the Purdue Campus data. Results show that MDLA and KMDLA can still obtain good classification results with the increasing noise in the image. Therefore, the two proposed algorithms can effectively reduce the noise of hyperspectral remote sensing images, improve the classification accuracy, and classify hyperspectral remote sensing images accurately.