下载中心
优秀审稿专家
优秀论文
相关链接
摘要
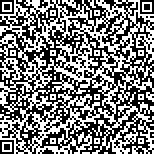
被动微波遥感土壤水分空间分辨率低,无法满足干旱监测、洪水预测以及灌溉管理等区域水利和农业等行业应用需求。中国在民用空间基础设施中规划论证的“陆地水资源卫星”搭载了雷达和辐射计主被动一体化微波载荷,通过主被动联合降尺度可以获取高分辨率(~5 km)的土壤水分,但其采用了一维合成孔径技术,主被动微波传感器观测的地面入射角是变化的,这给土壤水分反演及降尺度带来诸多挑战。本文从主被动微波遥感的物理机理和谱分析两种角度出发,利用闪电河流域的航空飞行试验数据,分析研究了基于主被动微波观测时间序列回归分析和基于谱分析的降尺度算法在辐射计和雷达入射角不同时的适用性。结果表明,在辐射计入射角度22.5°—27.5°时,基于主被动微波观测时间序列回归分析方法在27.5°时降尺度的结果最好,V极化和H极化的RMSE分别为7.57 K和7.46 K。基于谱分析方法在辐射计入射角度为22.5°和25°时得到的降尺度结果较好,V极化和H极化的最小RMSE分别为7.13 K和6.61 K,比基于主被动微波观测时间序列回归分析方法分别降低了0.44 K和0.85 K。基于主被动时间序列观测回归分析的降尺度方法,依赖于主被动微波观测的时间序列观测,当时序观测较短时,可能会因为回归分析的不稳定对降尺度结果造成较大的影响,而基于谱分析的降尺度方法则不需要依赖于长时间的时序观测。
Soil moisture derived from passive microwave remote sensing has a low spatial resolution, which cannot meet the requirement for the application of regional hydrological and agricultural activities, such as meteorological forecast, flood forecast, and irrigation management. The integrated microwave load of radar and radiometer loaded on “land-water resource satellite,” which is planned and demonstrated by Chinese civil space infrastructure, will obtain high-resolution soil moisture with the combination of active and passive microwave observation by using a one-dimensional synthetic aperture imaging observation mode. However, the incidence angle changes during observation. Present downscaling algorithms with combination of active and passive microwave observations are developed under the condition of fixed incident angles of radar and radiometer. In this study, downscaling algorithms based on time series regression analysis and spectral analysis are tested using the air flight experimental data of a lightning river basin to demonstrate that the feasibility of each for application to the incidence angles of radiometer and radar differs.The downscaling method based on time series regression analysis is the basic algorithm for Soil Moisture Active and Passive. The principal theoretical basis for this method is the linear relationship between the brightness temperature observation of radiometer and the backscatter coefficient observation of radar, and the linear relationship is related to surface roughness, vegetation, and incident angle. The spectral analysis downscaling method was first proposed and applied to remote sensing water vapor downscaling. Its theoretical basis is to obtain a high-resolution image by correctly simulating the spatial characteristics of the image spectral domain.The downscaling results based on active and passive observation regression analysis can reproduce spatial details, but the root-mean-square error (RMSE) is large. The minimum RMSE of V polarization is 7.57 K, and the minimum RMSE of H polarization is 7.46 K. The downscaling results based on spectral analysis can basically reflect the spatial distribution of the original observation. Nevertheless, evident plate phenomena occur in some areas, the spatial transition is not smooth, and the traces of downscaling are obvious. The minimum RMSE of H polarization is 7.13 K, and that of V polarization is 6.61 K. In accordance with the RMSE, the overall accuracy of the spectral downscaling method is higher than that of the time series regression analysis.The downscaling method based on the regression analysis of active and passive observation depends on the time series observation of active and passive microwave observation. The method of spectrum analysis does not need to regress to determine the relationship between active and passive microwave. It directly uses low-resolution passive radiometer observation and high-resolution radar copolarization (vv) observation for downscaling and does not need to rely on long-term time observation. The experimental results indicate that the time series regression analysis downscaling method can obtain the best results when the incident angle of radiometer is 27.5° and that of radar is 52.5° or 55°. The minimum RMSEs of V and H polarizations are 7.57 K and 7.46 K, respectively. The minimum RMSEs of V and H polarizations are 7.13 K and 6.61 K, respectively, for the spectral analysis downscaling method, which are 0.44k and 0.85 K, respectively, lower than those of the time series regression analysis downscaling method.