下载中心
优秀审稿专家
优秀论文
相关链接
摘要
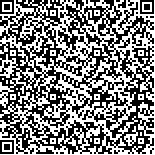
为了解决遥感卫星信息获取实效性差,严重依赖数据传输通信带宽,难以满足卫星在轨实时提取感兴趣目标需求等问题,本文设计了一种以全局统计为基础的高效舰船目标检测算法和超轻量化疑似目标真伪鉴别网络,实现舰船目标快速提取。采用传统图像处理方法快速检测目标,获取疑似目标切片,大幅降低数据量,采用自主设计改进的基于深度学习的超轻量鉴别网络实现疑似目标二次筛查,进一步提升目标提取精度。在算法模型实时实现过程中,合理优化算法流程和计算方法,建立计算精度误差分析模型,使得算法实时处理的精度、速度、硬件规模以及热耗等方面达到良好平衡。利用GF-3卫星数据对算法进行测试,试验表明该方法对复杂海洋背景环境、斑点噪声高、信噪比低的SAR遥感图像,舰船目标提取的准确性提升20%,达到98%,计算量降低90%,实时性提升50%。该方法兼顾了算法的有效性及在轨实时处理的可行性,可以在当前的星载嵌入式电路中实现并将在某新体制雷达试验卫星上在轨应用,具有良好的应用前景。
The low efficiency of orbit data processing and poor effectiveness of remote sensing satellite information acquisition are problems requiring great attention. Meeting the requirements of satellites in orbit in real time and efficient extraction of the targets of interest are challenging endeavors because of their heavy dependence on data transmission and communication bandwidth.In this paper, an efficient ship target detection algorithm based on global statistics and an ultra-lightweight suspected target identification network is designed to achieve the rapid extraction of ship targets. The traditional image processing method is used to detect the target quickly, obtain the suspected target slice, and reduce the amount of data significantly. A self-designed and improved ultra-lightweight identification network based on deep learning is then used to realize a second cycle of suspected target screening and improve the accuracy of target extraction. Much work has been done to simplify the real-time implementation of image-processing methods, such as OTSU threshold calculation, connected domain labeling, and target identification network based on deep learning. The real-time processing accuracy, speed, hardware scale, and heat consumption of the proposed method are well balanced by reasonable optimization of the algorithm flow and calculation method and establishment of an appropriate error analysis model. The calculation cost of the algorithm is low, and the dependence of the real-time implementation of the algorithm on the performance of the hardware platform is reduced greatly, thereby ensuring the excellent overall performance of the algorithm. In particular, network pruning, weight parameter sharing, and quantization are used to reduce the network weight parameter and forward reasoning calculation storage requirements, thus improving the design of the target identification network.GF-3 satellite data were used to test the algorithm, and experimental results showed that the accuracy of ship target extraction could be improved by 20%—98%, the computational complexity could be reduced by 90%, and the real-time performance could be improved by 50%. When the ship target extraction algorithm model was implemented in a low-power embedded circuit, the power consumption of the whole calculation circuit was less than 13 W, and the standby power consumption was less than 1.5 W; these values meet the requirements of full-time operations in orbit.The proposed method takes into account the effectiveness of the algorithm and feasibility of in-orbit real-time processing to improve the energy efficiency ratio and target extraction performance of the system effectively. The proposed algorithm was implemented in the current satellite embedded circuit and the orbit of a new radar test satellite, and the results obtained reflect good application prospects.