下载中心
优秀审稿专家
优秀论文
相关链接
摘要
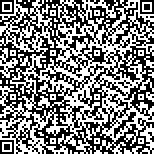
无人机遥感数据会衍生大量的光谱、纹理与结构特征,如何提取优势特征是提高红树林物种分类效率和精度的关键问题。针对深圳福田红树林自然保护区缓冲区获取的无人机高光谱影像和LiDAR点云数据,本研究旨在利用极端梯度提升算法(XGBoost)的“特征重要性”属性筛选出适合红树林物种分类的8类优势特征:基于无人机高光谱影像的单一特征(光谱波段、植被指数和纹理特征:F1—F3)及其融合特征(F4)、基于LiDAR点云的单一特征(高度和强度特征:F5和F6)及其融合特征(F7)、高光谱影像与LiDAR点云的融合特征(F8);基于以上优势特征构建8个XGBoost分类模型。结果表明:综合物种分类精度及其制图结果,基于F8特征的模型分类性能最佳(总体精度为96.41%,莫兰指数为0.5520);基于单一数据源融合特征(总体精度,F4:96.74%;F7:90.64%)的分类性能优于基于单一特征(总体精度,F1—F3:90.31%、92.20%和91.96%;F5和F6:87.66%和81.99%);基于融合特征(F4、F7和F8)和纹理特征(F3)分类图的莫兰指数比基于单一特征(F1、F2、F5和F6)的更大。本文论证了无人机遥感数据和XGBoost方法在基于像元的红树林物种精准分类上具备可行性,可为红树林生态系统健康、保护与恢复的立体监测提供科学依据和技术支撑。
Mangrove forest provides huge value of ecosystem services, such as beach protection, siltation promotion, flood and wave prevention, preventing waves, and biodiversity maintenance. Species composition and diversity are key parameters for assessing the health of forest ecosystem, and the loss of species diversity often accelerates degradation of structure and function of forest ecosystem. Therefore, accurately monitoring species composition and spatio-temporal distribution of mangrove forest are helpful for timely and effective management and restoration measures, which can further retain the quality of the mangrove ecosystem and biodiversity. The traditional means of obtaining mangrove species information requires time-consuming, labor-intensive and costly field survey, however, it is difficult to further understand the continuous distribution of forest health. In contrast, remote sensing technology is more cost-effective and can achieve spatially continuous monitoring of mangrove species composition and health status. With the fast-developing fine-resolution multispectral satellites, the images are used for classifying mangrove species due to their rich spatial geometric information. However, compared to multispectral images that contain limited spectral information, hyperspectral data are more effective for tree species discrimination or classification due to hundreds or even thousands of continuous bands that can reflect vegetation functional traits (e.g. pigment content, specific leaf area and nitrogen content). Moreover, LiDAR (Light Detection and Ranging) point cloud can acquire details related to three-dimensional features of the vegetation structure.Traditionally, there are many data dimension reduction or feature extraction methods, such as Principal Component Analysis (PCA), Independent Component Analysis (ICA), Minimum Noise Fraction (MNF) and Successive Projections Algorithm (SPA). However, these methods should combine with other classifiers in classification of plant species. To improve the classification accuracy and efficiency, this paper introduces a new machine learning method with both feature selection and classification functions—eXtreme Gradient Boosting (XGBoost). The rapidly growing Unmanned Aerial Vehicles (UAV) and portable sensors of hyperspectral imagery and LiDAR have provided higher quality remote sensing data. UAV-based remote sensing data can derive massive features of spectra, texture and structure, therefore, how to extract dominant features is a key issue to improve the efficiency and accuracy of mangrove species classification.With UAV-based hyperspectral imagery and LiDAR point cloud of the buffer area in Shenzhen Futian Mangrove Nature Reserve, this study aims to extract eight types of dominant features suitable for mangrove species classification using “feature_importance” property of XGBoost. The dominant features include: single feature derived from UAV-based hyperspectral imagery (spectral bands, vegetation indices and texture: F1—F3) and their fused feature (F4), single feature derived from UAV-based LiDAR point cloud (height and intensity feature: F5, F6) and their fused feature (F7), and fused feature coupling hyperspectral imagery and LiDAR point cloud.Synthetically considering species classification accuracy and mapping results, the classification model based on F8 feature held the best performance (overall accuracy was 96.41%, Moran’s I was 0.5520). The classification performance based on fused feature of single data source (F4 and F7, overall accuracy was separately 96.74% and 90.64%) was superior than that of single feature (F1—F3, F5 and F6, overall accuracy was 90.31%, 92.20%, 91.96%, 87.66% and 81.99% respectively). The Moran’s I of classification maps based on fused feature (F4, F7 and F8) and texture feature (F3) were greater than that of single feature (F1, F2, F5 and F6). Moreover, mangrove species classification models based on different dominant features have their own advantages on spatial mapping. The introduction of F3 effectively solved the common salt-and-pepper effect in the mapping results based on F1 and F2; moreover, the salt-and-pepper effect in the edge of classification images (near tidal flat area) was significantly improved in the mapping results based on F5, F7 and F8.We conclude that: (1) The combination of UAV-based remote sensing data and XGBoost is feasible to pixel-oriented accurate classification of mangrove species, the fusion feature of UAV-based hyperspectral image and LiDAR point cloud has the best classification effect when comprehensively comparing classification accuracy (OA) and mapping effect (Moran’s I); (2) when fusing the different types of features derived from UAV-based hyperspectral or LiDAR data alone, the corresponding classification accuracy and mapping effect behaved better than the single feature derived from the same UAV data source; (3) XGBoost, a machine learning method with both feature selection and classification functions, has great potential in remote sensing image classification; (4) the intensity features derived from LiDAR point clouds are greatly affected by UAV flight strips, however, the height features are robust in mangrove species classification. Future research will focus on unmixing of hyperspectral data, fuzzy classification and radiation transfer models (such as PROSAIL) to improve the accuracy and interpretability of mangrove species classification. This paper demonstrated the feasibility of UAV-based remote sensing data and XGBoost in the pixel-oriented precise classification of mangrove species, which can provide scientific basis and technical support for three-dimensional monitoring of health, conservation and restoration for mangrove ecosystem.