下载中心
优秀审稿专家
优秀论文
相关链接
摘要
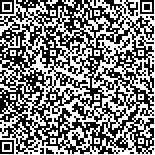
中国对地观测数据获取量持续增加,已步入遥感大数据时代,开展多星一体化数据处理与应用已成为发展趋势。文章从成像处理与信息提取两个方面系统回顾了技术发展过程,剖析了现有前沿方法的优势和特点,指出成像处理领域建立统一处理模型、信息提取领域建立高效学习模型面临的主要挑战。在此基础上,结合实际应用需求,提出了遥感大数据条件下多星一体化处理与分析的新思路,重点阐述了多星一体化处理与分析的基本概念、科学问题与解决方案,通过构建基于生成对抗网络的多星一体化成像处理模型与记忆保持的多任务特征共享与小样本增量学习模型,综合利用不同卫星和载荷间、不同任务和目标间信息,实现高质量图像产品的生成与高精度标注结果的提取。文末给出了技术途径和初步试验验证,并展望后续技术攻关方向。
With the rapid development of aerospace technology and the continuous increase of China’s high resolution earth observation data acquisition in the past few decades, the era of remote sensing big data has coming now. Developing multi-satellite integrated data processing and application technology has become an important trend. In this paper, we systematically review the technological development process from two aspects, including multi-satellite imaging processing and multi-element information extraction. Then, we analyze the advantages and characteristics of the existing cutting-edge methods, and points out the main challenge of establishing the integrated processing model for imaging processing field and efficient learning model for information extraction field. On this basis, as well as according to the practical application requirements, a novel method of multi-satellite integrated processing and analysis under remote sensing big data is proposed in this paper. We emphatically define the basic concepts, scientific problems, research ideas and solutions of this method. On one hand, in terms of multi-satellite imaging processing, aiming at the problem of difficult estimation of high-dimensional coupled imaging parameters at high resolution, the various errors caused by the whole cycles of electromagnetic waves are calculated quantitatively, including payload error, platform error, data transmission error, atmospheric influence and so on. Then a multi-satellite integrated imaging processing physical model based on the Generative Adversarial Networks (GAN) is constructed to approximate estimate imaging parameters. In this way, we can achieve high-precision geometric corrections and radiation corrections, as well as generate high-quality remote sensing image products. On the other hand, in terms of multi-element information extraction, to solve the problem that the accuracy of the original tasks is difficult to maintain due to the addition of new tasks and requires full sample retraining, we design a multi-task feature sharing model based on few-shot incremental learning. It has a novel memory retention unit and multi-modal joint optimization of convex non-negative matrix factors. Through this model, we can generate parallel and high-precision annotations for multiple objects. Compared with the existing methods, the information between different satellites and payloads, different missions and objects are complementary to each other, leading to the simultaneous improvement of multi-sensor imaging quality and object extraction accuracy. The specific technical approach and preliminary experimental verification are given in detail in this paper. Experiments on multi-satellite imaging processing show that our method can effectively estimate multiple imaging errors. The phase estimation accuracy is within 1 degree when the signal-to-noise ratio is -5dB, which indicates the good performance even under low signal-to-noise ratio condition. At the same time, experiments on multi-element information extraction show that compared to single-modal method, our method, which uses multi-modal data in combination and embedded the novel memory retention unit, improve the extraction accuracy in multi-tasks of object detection and semantic segmentation. In the future, we will pay more attention to the disconnection problem between multi-satellite imaging processing and multi-element information extraction in the field of remote sensing. By establishing a benign mutual feedback mechanism between these two procedures to maximize the benefits of the remote sensing big data.