下载中心
优秀审稿专家
优秀论文
相关链接
摘要
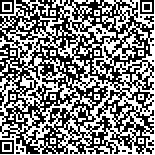
GF-6 WFV影像是中国首颗带有红边波段的中高分辨率8波段多光谱卫星的遥感影像,对于其影像及红边波段对作物分类影响的研究利用亟待展开。本文结合面向对象和深度学习提出一种适用于GF-6 WFV红边波段的卷积神经网络(RE-CNN)遥感影像作物分类方法。首先采用多尺度分割和ESP工具选择最佳分割参数完成影像分割,通过面向对象的CART决策树消除椒盐现象的同时提取植被区域,并转化为卷积神经网络的输入数据,最后基于Python和Numpy库构建的卷积神经网络模型(RE-CNN)用于影像作物分类及精度验证。有无红边波段的两组分类实验结果表明:在红边波段组,卷积神经网络(RE-CNN)作物分类识别取得了较好的效果,总体精度高达94.38%,相比无红边波段组分类精度提高了2.83%,验证了GF-6 WFV红边波段对作物分类的有效性。为GF-6 WFV红边波段影像用于作物的分类研究提供技术参考和借鉴价值。
The GF-6 WFV image is the first remote sensing image of the 8-band multi-spectral satellite with medium and high resolution in China. 4 spectrum bands including two red-edge bands are added to the image based on the conventional red, green, blue and near-infrared band. As a vegetation sensitive band, the red-edge band is one of the methods used for crop classification and identification in remote sensing images. Research on the impact of GF-6 WFV image and its red-edge bands on crop classification is urgently needed.This research uses GF-6 WFV images as the data source. The main work is (1) proposes a convolutional neural network (RE-CNN) remote sensing image crop classification model suitable for GF-6 WFV red-edge bands; (2) conducts crop classification research about GF-6 WFV imagery and its red-edge bands and evaluates effectiveness of red-edge bands due to the lack of relevant research, (3) uses the strategy of combining object-oriented and deep learning for crop classification. The core idea of this research: multi-scale segmentation is used in order to avoid the influence of salt and pepper phenomenon on image classification, and image segmentation is completed by selecting the best segmentation parameters with ESP tools and ROC-LV. Object-oriented classification by CART decision tree can extract vegetation area while eliminating salt and pepper noise, and convert it into input data of convolutional neural network. The network structure of Inception was introduced to extract the multi-scale features of the image and then a convolutional neural network model (RE-CNN) for GF-6 WFV imagery was constructed for crop classification. A control experimental group with or without red-edge bands was set up and the RE-CNN model was used for crop classification and accuracy verification. The effect of the newly added red-edge bands on crop classification is studied, and the effectiveness and sensitivity of the red-edge band are evaluated in crop classification by GF-6 WFV imagery.The experimental results of this study show that: (1) Object-oriented CART decision tree classification effectively eliminates salt and pepper noise in vegetation area extraction, and the classification strategy of combining with deep learning achieve better classification results in remote sensing image crop classification. (2) The RE-CNN model proposed in this paper can be used for GF-6 WFV remote sensing image crop classification. The classification accuracy of the experimental group in the group of red-edge bands is as high as 94.38%, and the Kappa coefficient is 0.92. (3) The newly added red-edge bands in GF-6 WFV images can effectively improve the crop classification accuracy of remote sensing image. Compared with the group without red-edge bands, the classification accuracy is increased by 2.83%, which verifies the effectiveness and sensitivity that the newly-added red-edge bands in GF-6 WFV images improves the classification accuracy. Moreover, it provides a reference for the research of GF-6 WFV image and its red-edge band for crop classification.