下载中心
优秀审稿专家
优秀论文
相关链接
摘要
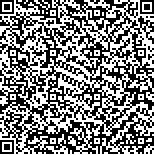
高空间、高时间分辨率的遥感影像对地表与大气环境的实时精细监测具有重要作用,但单一卫星传感器获取的遥感影像存在空间与时间分辨率相互制约的问题,时空融合技术发展成为了低成本、高效生成满足不同应用需求的高时空分辨率遥感影像的有效手段。近年来,国内外学者提出了大量的时空融合算法,但对于复杂的地物类型变化的空间细节修复仍存在挑战,融合影像精度有待提高。对此,本文提出增强型空间像元分解时空遥感影像融合算法(EUSTFM),采用变化检测识别并修复地物类型改变的像元,使空间像元分解过程可同时在已知时相与未知时相进行,以生成空间细节信息准确的中间分辨率影像对,用于最终的邻域相似像元计算,实现了对季节性变化(如植被自然生长)、有形变(如城市土地扩张)及无形变的地物类型变化(如农作物的成熟与收割)等复杂地表变化的一致性预测,提高了融合精度。实验采用两对Landsat-MODIS遥感影像数据集,对比STARFM与FSDAF两种广泛应用的时空融合算法,测试了该算法的影像融合效果。结果表明,本文提出的EUSTFM能够同时实现对季节性变化及复杂的地物类型变化的稳定预测,可生成具有更高精度的融合影像,将有效推动时空影像融合的实际遥感应用。
Remote sensing images with high spatial and temporal resolutions are vital for the real-time and fine monitoring of land surface and atmospheric environment. However, a single satellite sensor has to tradeoff between the spatial and temporal resolutions due to technical and budget limitations. In recent years, numerous spatial and temporal image fusion models have been proposed to produce high-resolution images with low cost and remarkable effectiveness. Despite the varying levels of success in the accuracy of fused images and the efficiency of algorithms, challenges always remain on the recovery of spatial details along with the complex land cover changes. This study presented an enhanced unmixing model for spatial and temporal image fusion (EUSTFM) that accounts for phenological changes (e.g., vegetation growth) and shape (e.g., urban expansion) and non-shape land cover changes (e.g., crop rotation) on the land surface simultaneously. First, a change detection method was devised to identify the pixels with land cover change. The similar pixels of the detected pixels were then searched in the neighborhood to recompose the spectral reflectance on the prediction date. Thus, the real land cover class on the prediction date can be defined using the recomposed high-resolution image rather than directly using the classification result from a prior date. Subsequently, the spatial unmixing of pixels can be conducted on the prior and prediction dates to produce a medium-resolution image pair with accurate spatial details. Finally, the calculation of the similar pixels in the neighborhood was implemented for the final prediction of the fused images using all the original high and low-resolution image pair in the prior time, low-resolution image in the prediction time, and the produced medium-resolution image pair in the prior and prediction times. This study tested the algorithms with two actual Landsat-MODIS datasets: one dataset focusing on typical phenological changes in a complex landscape in Australia and the other dataset focusing on shape land cover changes in Shenzhen, China, to demonstrate the performance of the proposed EUSTFM for complex temporal changes on various landscapes. Comparisons with the popular spatiotemporal fusion models, including Spatial and Temporal Adaptive Reference Fusion Model (STARFM) and Flexible Spatiotemporal DAta Fusion (FSDAF), showed that EUSTFM can robustly achieve a better fusion accuracy for all the phenological, non-shape, and shape land cover changes. The fused results using STARFM and FSDAF showed significant differences between the green band and the two other bands for typical phenological changes on a complex landscape in Australia. By contrast, the fused images using EUSTFM showed consistently high accuracy in all the three bands. This finding revealed a better performance for the fusion of images with various spatial resolution gaps, including a factor of 8 in near-infrared and red bands and a factor of 16 in the green bands. The proposed EUSTFM shows great potential in facilitating the monitoring of complex and diverse land surface dynamics.